December 06, 2014
Using LaTeX for a paper for Science Advances
I recently wrote a paper for the new AAAS journal Science Advances using LaTeX (as opposed to their Microsoft Word template), and have some things to share with others interested in sending their beautifully typeset work to that journal. [1]
First, Science Advances uses a bibliography style that is slightly different from that of Science, which means that the Science.bst file available from AAAS for submissions to Science is not suitable. Specifically, Science Advances wants full titles to be listed and wants full page ranges (rather than just the first page). My reading of the detailed information for authors suggests that these are the only differences. Here is a modified version of the Science.bst file, called ScienceAdvances.bst, that conforms to the required bibliographic style. [2]
Second, Science Advances uses a slightly different format for the manuscript itself than Science, and so again, the existing LaTeX template is not quite suitable. One difference is that Science Advances requires section headings. Here is a zip file containing a Science Advances LaTeX template, modified from the Science template distributed by AAAS, that you can use (note: this zip includes the bst file listed above). [2]
Finally, there are a few little things that make Science Advances different from Science. SA has a much longer (effective) length limit, being 15,000 words compared to Science's 4500 words. The Reference list in SA is comprehensive, meaning that references cited only in the Supplementary Material should be included in the main text's reference list. There is also no limit on the number of references (compared to Science's limit of 40). And, SA places the acknowledgements after the Reference section, and the acknowledgements include information about funding, contributions, and conflicts of interest. Otherwise, the overall emphasis on articles being of broad interest across the sciences and of being written in plain English [3] remains the same as Science.
-----
[1] Full disclosure: I am currently serving as an Associate Editor for Science Advances. Adapting Science's LaTeX files to Science Advances's requirements, and sharing them online, was not a part of my duties as an AE.
[2] The files are provided as-is, with no guarantees. They compile for me, which was good enough at the time.
[3] Of course, biology articles in Science are hardly written in "plain English", so there is definitely some degree of a double-standard at AAAS for biology vs. non-biology articles. Often, it seems that biology, and particularly molecular biology, can be written in dense jargon, while non-biology, but especially anything with mathematical concepts or quantities in it, has to be written without jargon. This is almost surely related to the fact that the majority of articles published in Science (apparently by design) are biomedical in nature. AAAS is claiming that Science Advances will be different, having a broader scope and a greater representation of non-biomedical articles (for instance, SA specifically says it wants articles from the social sciences, the computer sciences, and engineering, which I think is a really great stance). Whether they can pull that off remains to be seen, since they need to get the buy-in from the best people in these other fields to send their high-quality work to SA rather than to disciplinary venues.
posted December 6, 2014 03:15 PM in Simply Academic | permalink | Comments (0)
December 03, 2014
Grants and fundraising (Advice to young scholars, part 4 of 4)
These notes are an adapted summary of the the 4th of 4 professional development panels for young scholars, as part of the American Mathematical Society (AMS) Mathematics Research Community (MRC) on Network Science, held in June 2014. Their focus was on mathematics, computer science, and networks, but many of the comments generalize to other fields. [1,2]
Panel 4. Grants and Fundraising
Opening remarks: In general, only around 10% of grant proposals are successful. But, roughly 60% of submitted proposals are crap. Your competition for getting funded is the non-crappy 40%. Therefore, work hard to polish your proposals, and take as much time as you would a serious or flagship paper. Get feedback from colleagues on your proposals before submitting, and try as hard as possible to get that feedback at least one month before the deadline. (Many institutions have these "mock panels" available, and they are incredibly useful, especially for early career scientists.) Practice makes the master, so consider writing a grant proposal as a postdoc. Having some success as a postdoc will also make you look more attractive as a faculty candidate. Know when the annual deadlines are for the regular grant competitions, and plan ahead. Try to avoid the last-minute crush of writing proposals in two weeks or less.
- What should be in a proposal?
Really exciting research. But, try to propose to do more than just really exciting research. Consider organizing workshops, creating new classes, creating notes, giving public lectures, hosting undergraduates, working with underrepresented groups, running a podcast series, and even teaching in a local high school. - What kinds of proposals should an early-career person write?
In your first few years as faculty, apply to all the early-career fellowships and competitions that you can comfortably manage. That includes the Sloan, McDonnell, Packard, etc., along with the NSF CAREER award, and the various "early investigator" competitions at the DoD and other places. Figure out what people do in your field and do that too. These awards are sometimes for sizable amounts of funding, but even if they are not, they are often very prestigious. - How many grants do I need?
This depends on the size of your preferred research group. Many faculty try to keep 2-3 active grants at once, and write approximately 1-2 new proposals per year. As a rough calculation, a "normal sized" grant from many parts of NSF will support 1 graduate student for its duration (plus modest summer salary, travel, and computing equipment). - Can I propose work that I have already partially completed?
Yes. This is common, and often even recommended. "Preliminary results" make a proposal sound less risky, and basically the reviewers are looking for proposals that are exciting, will advance the state-of-the-art, well written, and exceedingly likely to succeed. If you've already worked out many of the details of the work itself, it is much easier to write a compelling proposal. - Proposals are often required to be understandable by a broad audience but also include technical details, so how do you balance these requirements?
An advanced undergraduate should be able to understand your proposal with some training. Most panels have some experts who can judge the technical details. A good strategy for learning how to balance technical material versus accessibility is to read other people's proposals, especially successful ones, even outside your field. The first pages of any proposal should be more broadly understandable, while the final pages may be decodable by experts only. - Can you reuse the same material for multiple grants?
It's best not to double dip. If a grant is rejected, you can usually resubmit it, often to the same agency (although sometimes not more than once). Because you have some feedback and you have already written the first proposal, it's often less work to revise and resubmit a rejected proposal. (But, the goal posts may move with the resubmission because the review panel may be composed of different people with different opinions, e.g., at NSF.) Small amounts of overlap are usually okay, but if you don't have anything new to propose, don't submit a proposal.
Pro tips:
- Calls For Proposals (CFPs) are often difficult to decode, so don't hesitate to ask for help to translate, either from your colleagues or from the cognizant program officer. Usually, the specific words and pitch of a program have been shaped by other researchers' interests, and knowing what those words really mean can help in deciding if your ideas are a good match for the program.
- Proposals are reviewed differently depending on the agency. NSF proposals are reviewed by ad hoc committees of practicing scientists (drawn essentially at random from a particular broad domain). NIH proposals are reviewed by study panels whose membership is fairly stable over time. DoD proposals are reviewed internally, but sometimes with input from outside individuals (who may or may not be academics).
- Don't write the budget yourself. Use the resources of your department. You will eventually learn many things about budgeting, but your time is better spent writing about the science. That being said, you will need to think about budgets a lot because they are what pay for the research to get done (and universities and funding agencies really love to treat them like immutable, sacred documents). Familiarize yourself with the actual expenses associated with your kind of research, and with the projects that you currently and aim to do in the future.
- For NSF, don't budget for funding to support undergraduates during the summer; instead, assume that you will apply for (and receive) an REU Supplement to your award to cover them. The funding rate for these is well above 50%.
- NSF (and some other agencies) have byzantine rules about the structure, format, and set of documents included in a proposal. They now routinely reject without review proposals that don't follow these rules to the letter. Don't be one of those people.
- Ending up with leftover money is not good. Write an accurate budget and spend it. Many agencies (e.g., NSF and NIH) will allow you to do a 1-year "no cost extension" to spend the remaining money.
- Program officers at NSF are typically professors, on leave for 2-3 years, so speak to them at conferences. Program officers at DoD agencies and private foundations are typically professionals (not academics). NSF program officers exert fairly little influence over the review and scoring process of proposals. DoD and foundation program officers exert enormous influence over their process.
-----
[1] Panelists were Mason Porter (Oxford), David Kempe (Southern California), and me (the MRC organizers), along with an ad hoc assortment of individuals from the MRC itself, as per their expertise. The notes were compiled by MRC participants, and I then edited and expanded upon them for clarity and completeness, and to remove identifying information. Notes made public with permission.
[2] Here is a complete copy of the notes for all four panels (PDF).
posted December 3, 2014 03:46 AM in Simply Academic | permalink | Comments (0)
December 02, 2014
Doing interdisciplinary work (Advice to young scholars, part 3 of 4)
These notes are an adapted summary of the the 3rd of 4 professional development panels for young scholars, as part of the American Mathematical Society (AMS) Mathematics Research Community (MRC) on Network Science, held in June 2014. Their focus was on mathematics, computer science, and networks, but many of the comments generalize to other fields. [1]
Panel 3. Interdisciplinary Research
Opening remarks: Sometimes, the most interesting problems come from interdisciplinary fields, and interdisciplinary researchers are becoming more and more common. As network scientists, we tend to fit in with many disciplines. That said, the most important thing you have is time; therefore, choose your collaborations wisely. Interdisciplinary work can be divided into collaboration and publication, and each of these has its own set of difficulties. A common experience with interdisciplinary work is this:
Any paper that aims for the union of two fields will appeal mainly to the intersection. -- Jon Kleinberg
- What's the deal with interdisciplinary collaborations? How do they impact your academic reputation?
There are three main points to consider when choosing interdisciplinary collaborations, and how they impact perceptions of your academic reputation.
First, academia is very tribal, and the opinions of these tribes with regards to your work can have a huge impact on your career. Some departments won't value work outside their scope. (Some even have a short list of sanctioned publication venues, with work outside these venues counting literally as zero for your assessments.) Other departments are more open minded. In general, it's important to signal to your hopefully-future-colleagues that you are "one of them." This can mean publishing in certain places, or working on certain classes of problems, or using certain language in your work, etc. If you value interdisciplinary work, then you want to end up in a department that also values it.
Second, it's strategically advantageous to be "the person who is the expert on X," where X might be algorithms or statistics or models for networks, or whatever. Your research specialty won't necessarily align completely with any particular department, but it should align well with a particular external research community. In the long run, it is much more important to fit into your community than to fit into your department, research-wise. This community will be the group of people who review your papers, who write your external letters when you go up for tenure, who review your grant proposals, who hire your students as postdocs, etc. The worst possible situation is to be community-less. You don't have to choose your community now, but it helps to choose far enough ahead of your tenure case that you have time to build a strong reputation with them.
Third, make sure the research is interesting to you. If your contribution in some interdisciplinary collaboration is to point out that an off-the-shelf algorithm solves the problem at hand, it's probably not interesting to you, even if it's very interesting to the collaborator. Even if it gives you an easy publication, it won't have much value to your reputation in your community. Your work will be compared to the work of people who do only one type of research in both fields, and might not look particularly good to any field.
Be very careful about potentially complicated collaborations in the early stages of your career. Be noncommittal until you're sure that your personalities and tastes in problems match. (Getting "divorced" from a collaborator, once a project has started, can be exhausting and complicated.) Being able to recognize cultural differences is an important first step to good collaborations, and moving forward effectively. Don't burn bridges, but don't fall into the trap of saying yes to too many things. Be open to writing for an audience that is not your primary research community, and be open to learning what makes an interesting question and a satisfying answer in another field. - What's the deal with publishing interdisciplinary work? Where should it go?
As a mathematical or computer or data scientist doing work in a domain, be sure to engage with that domain's community. This helps ensure that you're doing relevant good work, and not reinventing wheels. Attend talks at other departments at your university, attend workshops/conferences in the domain, and discuss your results with people in the domain audience.
When writing, vocabulary is important. Knowing how to speak another discipline's language will help you write in a way that satisfies reviewers from that community. Less cynically, it also helps the audience of that journal understand your results, which is the real goal. If publishing in the arena of a collaborator, trust your collaborator on the language/writing style.
In general, know what part of the paper is the most interesting, e.g., the mathematics, or the method or algorithm, or the application and relationship to scientific hypotheses, etc., and send the paper to a venue that primarily values that thing. This can sometimes be difficult, since academic tribes are, by their nature, fairly conservative, and attempting to publish a new or interdisciplinary idea can meet with knee-jerk resistance. Interdisciplinary journals like PLOS ONE, which try not to consider domain, can be an okay solution for early work that has trouble finding a home. But, don't overuse these venues, since they tend also to not have a community of readers built in the way regular venues do.
Note: When you interview for a faculty position, among the many questions that you should be asking the interviewing department: "In practice, how is your department interdisciplinary? How do you consider interdisciplinary work when evaluating young faculty (e.g., at tenure time)?"
-----
[1] Panelists were Mason Porter (Oxford), David Kempe (Southern California), and me (the MRC organizers), along with an ad hoc assortment of individuals from the MRC itself, as per their expertise. The notes were compiled by MRC participants, and I then edited and expanded upon them for clarity and completeness, and to remove identifying information. Notes made public with permission.
[2] Here is a complete copy of the notes for all four panels (PDF).
posted December 2, 2014 03:40 AM in Simply Academic | permalink | Comments (0)
December 01, 2014
Balancing work and life (Advice to young scholars, part 2 of 4)
These notes are an adapted summary of the the 2nd of 4 professional development panels for young scholars, as part of the American Mathematical Society (AMS) Mathematics Research Community (MRC) on Network Science, held in June 2014. Their focus was on mathematics, computer science, and networks, but many of the comments generalize to other fields. [1,2]
Panel 2. Life / Work Balance
Opening remarks: "Academia is like art because we're all a little crazy."
Productivity often scales with time spent. A good strategy is to find enough of a balance so that you don't implode or burn out or become bitter. The best way to find that balance is to experiment! Social norms in academia are slowly shifting to be more sensitive about work/life balance issues, but academia changes slowly and sometimes you will feel judged. Often, those judging are senior faculty, possibly because of classical gender roles in the family and the fact that their children (if any) are usually grown. Telling people you're unavailable is uncomfortable, but you will get used to it. Pressure will be constant, so if you want a life and/or a family, you just have to do it. Routines can be powerful--make some rules about when your non-work hours are during the week and stick to them.
- What if I want to have children?
Most institutions have a standard paternity/maternity leave option: one semester off of research/teaching/service plus a one-year pause on your tenure clock. If you think you will have children while being faculty, ask about the parental leave policy during your job interview. Faculty with small children often have to deal with scheduling constraints driven by day care hours, or at-home responsibilities for child care; they are often simply unavailable nights and evenings, so be sensitive to that (don't assume they will be available for work stuff then). Juggling a brand new faculty job and a new baby in the same year can be done, but it can also burn you out. - Burnout, what?
It's hard to get numbers on burnout rate, in part because there are varying degrees of ``burnout'' and different people burn out in different ways. Most tenured faculty are not completely burned out; true burnout often turns into leaving academia. On the other hand, some faculty have real breakdowns and then get back on the horse. Other faculty give up on the ``rat race'' of fundraising and publishing in highly competitive venues and instead focus on teaching or service. There are many ways to stop being productive and lose the passion.
One strategy is to promise yourself that once it stops being fun, leave and go get a satisfying 9-5 job (that pays better). - What about all this service stuff I'm getting asked to do?
Service (to your department, to your university, and to your research community) is an important part of being a professor. You will get asked to do many things, many of which you've never done before, some of which will sound exciting. As an early-career person, you should learn to say "no" to things and feel comfortable with that decision. Until you have tenure, it's okay to be fairly selfish about your service--think about whether saying "yes" will have a benefit to your own research efforts. If the benefit is marginal, then you should probably say no.
There are a lot of factors that go into whether or not you say yes to something. It's important to learn to tell the difference between something you should say no or yes to. A key part of this is having one or more senior faculty mentors you can ask. Ideally, have one inside your department and one outside your department but within your research community. - What happens during summers?
If you're willing to set yourself up for it, then you can readily take a month-long vacation with absolutely no contact. Tell your department head that you're not bringing your laptop. That being said, summer is often the time where many faculty try to focus exclusively on research, since they're not teaching. At most institutions, it's normal for regular departmental committees to not meet, so you often get a break from your departmental service obligations then, too. - How many hours should I work each week?
How much you work each week is really up to you. Some people work 80-85 hours during terms, and 70 between terms. A common number kicked around is 60, and relatively few people work a lot less than that. For the most part, faculty work these hours by choice. The great advantage of faculty life is that your schedule is pretty flexible, which allows you to carve out specific time for other things (e.g., life / family). Many faculty work 9-5 on campus, and then add other hours at home or otherwise off campus. Some others work long hours during the week and then are offline on the weekends. - Do I have to spend all those hours on campus?
If you don't get "face time" with your institution and the people evaluating your tenure case, then they will form negative opinions about you. So go into work often. And, spend time "in your lab," with your students. Good idea to have lunch with every one of your fellow tenure-track faculty during your early faculty career. - I have a two body problem.
Solving the two-body problem (marriage with another academic or other professional career type) can be tricky. Start talking about it with your partner long before you start applying to jobs. One solution: make a list and let your partner cross off the things that don't make sense. In job negotiations, there are things that the department can do, such as interview/hire your spouse (or encourage/fund another department to do so). If your partner is not an academic there are few things the university can do, but often the more senior people have contacts and that can help.
One strategy is to always go for the interview, get the offer first, and think about it later. Departments often want to know ahead of time whether they'll need to help with the two-body problem in order to get you to say yes. (But, they are legally not allowed to ask you if you have a partner, so you have to bring it up.) This can (but not necessarily) hurt your offer. Also, when women interview, they get assumptions imposed on them, such as the existence of a two-body problem. Some women don't wear a wedding ring to an interview in order to avoid those assumptions. One possibility is to consider saying something in advance along the lines of ``my husband is excited and there's no problem.’’ - How much should I travel?
Many strategies. Mostly depends on your personal preferences. A popular strategy is to travel no more than once a month. Also consider picking trips on which you can bring your family and/or do some extra traveling. As a junior person, however, traveling is in part about reputation-building, and is a necessary part of academic success.
-----
[1] Panelists were Mason Porter (Oxford), David Kempe (Southern California), and me (the MRC organizers), along with an ad hoc assortment of individuals from the MRC itself, as per their expertise. The notes were compiled by MRC participants, and I then edited and expanded upon them for clarity and completeness, and to remove identifying information. Notes made public with permission.
[2] Here is a complete copy of the notes for all four panels (PDF).
posted December 1, 2014 03:25 AM in Simply Academic | permalink | Comments (0)
November 27, 2014
The faculty market (Advice to young scholars, part 1 of 4)
These notes are an adapted summary of the the 1st of 4 professional development panels for young scholars, as part of the American Mathematical Society (AMS) Mathematics Research Community (MRC) on Network Science, held in June 2014. Their focus was on mathematics, computer science, and networks, but many of the comments generalize to other fields. [1,2]
Panel 1. The Academic Job Market
Opening remarks: The faculty hiring process is much more personal than the process to get into grad school. Those who are interviewing you are evaluating whether you should be a coworker for the rest of their careers! The single most-important thing about preparing to apply for faculty jobs is to have a strong CV for the type of job you're applying for. If you're on the tenure-track, that nearly always means being able to show good research productivity for your field (publications) and having them be published in the right places for your field.
- Where did you find job postings? Where did you search?
It depends on the type of job and the field. For math: AMS weekly mailings, back of SIAM news. For physics: the back of Physics Today. For computer science: CRA.org/jobs, Communications of the ACM. For liberal arts colleges: chronicle vitae. In general: mathjobs.org, academicjobs.org, and ask your supervisor(s) or coauthors. - When do you apply?
The U.S. market is, for the most part, seasonal. The seasonality differs by field. Biology searches may start in September, with interviews in November and December. Math and computer science tend to have applications due in November, December, and maybe even January. In the U.K., institutions tend to hire whenever, regardless of season. Timing for interdisciplinary positions may be a little strange. It is worth figuring out 6 months ahead of time what the usual timeline is for your field. - What kind of department should you apply to?
If you're in department X, you will be expected to teach courses in department X. (At most institutions, you will teach a mixture of undergraduate and graduate-level courses, but not always within your research speciality.) It may be better to have your publications match the departments to which you apply; for instance, if you're interested in jobs in math departments, you should be publishing in the SIAM journals. You should also get letter writers in that field, since their name will be more recognizable to the hiring committee (and thus carry more weight). - What should you put in a cover letter?
The cover letter is the first (and sometimes only) thing the hiring committee sees. In some fields, the cover letter is 1 full page of text and serves as a complete abstract of your application packet (i.e., it describes your preparation, major research areas and achievements, and intended future research agenda). If you have a specific interest in a department / location, say it in the cover letter (e.g., "I have family living in X and want to be close to them") since this signals to the hiring committee that you're genuinely interested in their institution. Also, mention the people in the department whom you would like to look at your application. Mention a few specific things about the individual advertisement (no one likes to feel spammed). Finally, the cover letter is your one chance to explain anything that might look like a red flag to the committee. - What should you put in a teaching statement?
At research universities, teaching statements are usually the last thing that is read. For junior-level positions, their contents often cannot help your changes, but a bad statement can hurt them. At liberal arts / teaching colleges, a compelling teaching statement is very important. - What about letters of recommendation?
Letters of recommendation are the second most important thing in your packet (the most important being your publication record). The best letters are those that can state firmly that you are in the top whatever percent of students or postdocs. Their description of you is the most important, and their own fame is second. There are some cultural differences between the U.K., U.S., and other places in terms of how glowing they will be. An excited letter from an unknown writer is more important than a mediocre letter from a famous person. The absence of a letter from a PhD or postdoc advisor will be interpreted as a red flag. - Are software and blogs good or bad?
Sometimes good, sometimes not. Don't do these things at the cost of your own research. If you have specific reasons for doing these things, emphasize them in your research statement as "sweeteners" to your strong publication record. For tenure-track faculty jobs, these things generally cannot compensate for a poor or mediocre publication record. The research itself is the most important thing. - How does the hiring committee work?
At most institutions today, the ratio of candidates to faculty jobs is roughly 100:1. At major research institutions, about 60% of those candidates are not competitive to begin with; it's the other 40% you have to beat. This means the hiring committee has an enormous job to do just to narrow the pool to the 10-20% or so that they'll scrutinize closely. Your goal is to make it into that group, so that your star qualities can be properly noticed.
A common strategy that hiring committees take is to progressively pay more attention to a progressively smaller pool. Your goal is to get through the first few waves of filtering until you can get a serious look by the committee. Two very common reasons a candidate is dropped from the pool during these early evaluations are (i) their area of research is not a good match to the areas of interest in the search (including not looking like the kind of candidate the committee thinks they want, e.g., because their work appears in unusual places), and (ii) their research productivity is not good enough (usually controlled for time since PhD). Both are subjective criteria and vary by search. In general, the more prestigious your PhD, the more prestigious your publication venues, and the more prestigious your letter writers, the better you will fare. - What about the interview itself?
Usually 1-2 days of intense, back-to-back meetings with faculty, plus a meeting with a group of graduate students, plus 1-2 dinners with faculty, plus a job talk (about your research), and sometimes also a "teaching talk." In your job talk, you need to convince them of why they should hire someone doing exactly the research you're doing. Make the audience excited. Make it related to things they know about. Be sure to look at the webpage of every person that might be in the room. Be sure to ask for your meeting schedule in advance, and then read up a little about each person you will meet.
Pro Tips:
- "Exploding offers" (offers that expire after a few weeks) may be used by lower-tier institutions when trying to hire a person likely to have offers from higher-tier institutions. But, deadlines are often negotiable. Play nice. It's often not malicious, but rather just to proceed quickly down the ranked list of candidates. Moreover, if you turn it down in a friendly conversation, you may be able to negotiate a "if you are still interested in me in a month, please let me know."
- During the year before you apply, figure out what departments you'll be applying to, and be sure to have some publications and talks at major conferences for that type of field or department.
- Don't pad your CV. Put all preprint and in-prep publications in a separate, clearly-labelled section. CV readers will look at your PhD, your research interests, and then your publications. Awards (e.g. Best Paper) and high quality venues are more important than quantity.
- You could email people at the target department(s) saying "Here's a paper, btw: I'll be applying soon." If you're uncomfortable with that, your advisor could do it.
- If you are applying to a lower tier school than your pedigree, tailor the application well. You must send a very strong signal that you are serious. (Otherwise, they may not even interview you.)
-----
[1] Panelists were Mason Porter (Oxford), David Kempe (Southern California), and me (the MRC organizers), along with an ad hoc assortment of individuals from the MRC itself, as per their expertise. The notes were compiled by MRC participants, and I then edited and expanded upon them for clarity and completeness, and to remove identifying information. Notes made public with permission.
[2] Here is a complete copy of the notes for all four panels (PDF).
posted November 27, 2014 03:04 AM in Simply Academic | permalink | Comments (1)
January 29, 2014
PLOS mandates data availability. Is this a good thing?
The Public Library of Science, aka PLOS, recently announced a new policy on the availability of the data used in all papers published in all PLOS journals. The mandate is simple: all data must be either already publicly available, or be made publicly available before publication, except under certain circumstances [1].
On the face of it, this is fantastic news. It is wholly in line with PLOS’s larger mission of making the outcomes of science open to all, and supports the morally correct goal of making all scientific knowledge accessible to every human. It should also help preserve data for posterity, as apparently a paper’s underlying data becomes increasingly hard to find as the paper ages [2]. But, I think the the truth is more complicated.
PLOS claims that it has always encouraged authors to make their data publicly available, and I imagine that in the vast majority of cases, those data are in fact available. But the policy does change two things: (i) data availability is now a requirement for publication, and (ii) the data are supposed to be deposited in a third-party repository that makes them available without restriction or attached to the paper as supplementary files. The first part ensures that authors who would previously decline or ignore the request for open data must now fall into line. The second part means that a mere promise by the authors to share the data with others is now insufficient. It is the second part where things get complicated, and the first part is meaningless without practical solutions to the second part.
First, the argument for wanting all data associated with scientific papers to be publicly available is a good one, and I think it is also the right one. If scientific papers are in the public domain [3], but the data underlying their results are not, then have we really advanced human knowledge? In fact, it depends on what kind of knowledge the paper is claiming to have produced. If the knowledge is purely conceptual or mathematical, then the important parts are already contained in the paper itself. This situation covers only a smallish fraction of papers. The vast majority report figures, tables or values derived from empirical data, taken from an experiment or an observational study. If those underlying data are not available to others, then the claims in the paper cannot be exactly replicated.
Some people argue that if the data are unavailable, then the claims of a paper cannot be evaluated at all, but that is naive. Sometimes it is crucial to use exactly the same data, for instance, if you are trying to understand whether the authors made a mistake, whether the data are corrupted in some way, or understand a particular method. For these efforts, data availability is clearly helpful.
But, science aspires for general knowledge and understanding, and thus getting results using different data of the same type but which are still consistent with the original claims is actually a larger step forward than simply following exactly the same steps of the original paper. Making all data available may thus have an unintended consequence of reducing the amount of time scientists spend trying to generalize, because it will be easier and faster to simply reuse the existing data rather than work out how to collect a new, slightly different data set or understand the details that went into collecting the original data in the first place. As a result, data availability is likely to increase the rate at which erroneous claims are published. In fields like network science, this kind of data reuse is the norm, and thus gives us some guidance about what kinds of issues other fields might encounter as data sharing becomes more common [4].
Of course, reusing existing data really does have genuine benefits, and in most cases these almost surely outweigh the more nebulous costs I just described. For instance, data availability means that errors can be more quickly identified because we can look at the original data to find them. Science is usually self-correcting anyway, but having the original data available is likely to increase the rate at which erroneous claims are identified and corrected [5]. And, perhaps more importantly, other scientists can use the original data in ways that the original authors did not imagine.
Second, and more critically for PLOS’s new policy, there are practical problems associated with passing research data to a third party for storage. The first problem is deciding who counts as an acceptable third party. If there is any lesson from the Internet age, it is that third parties have a tendency to disappear, in the long run, taking all of their data with them [6]. This is true both for private and public entities, as continued existence depends on continued funding, and continued funding, when that funding comes from users or the government, is a big assumption. For instance, the National Science Foundation is responsible for funding the first few years of many centers and institutes, but NSF makes it a policy to make few or no long-term commitments on the time scales PLOS’s policy assumes. Who then should qualify as a third party? In my mind, there is only one possibility: university libraries, who already have a mandate to preserve knowledge, should be tapped to also store the data associated with the papers they already store. I can think of no other type of entity with as long a time horizon, as stable a funding horizon, and as strong a mandate for doing exactly this thing. PLOS’s policy does not suggest that libraries are an acceptable repository (perhaps because libraries themselves fulfill this role only rarely right now), and only provides the vague guidance that authors should follow the standards of their field and choose a reasonable third party. This kind of statement seems fine for fields with well-developed standards, but it will likely generate enormous confusion in all other fields.
This brings us to another major problem with the storage of research data. Most data sets are small enough to be included as supplementary files associated with the paper, and this seems right and reasonable. But, some data sets are really big, and these pose special problems. For instance, last year I published an open access paper in Scientific Reports that used a 20TB data set of scoring dynamics in a massive online game. Data sets of that scale might be uncommon today, but they still pose a real logistical problem for passing it to a third party for storage and access. If someone requests a copy of the entire data set, who pays for the stack of hard drives required to send it to them? What happens when the third party has hundreds or thousands of such data sets, and receives dozens or more requests per day? These are questions that the scientific community is still trying to answer. Again, PLOS’s policy only pays lip service to this issue, saying that authors should contact PLOS for guidance on “datasets too large for sharing via repositories.”
The final major problem is that not all data should be shared. For instance, data from human-subjects research often includes sensitive information about the participants, e.g., names, addresses, private behavior, etc., and it is unethical to share such data [7]. PLOS’s policy explicitly covers this concern, saying that data on humans must adhere to the existing rules about preserving privacy, etc.
But what about large data sets on human behavior, such as mobile phone call records? These data sets promise to shed new light on human behavior of many kinds and help us understand how entire populations behave, but should these data sets be made publicly available? I am not sure. Research has shown, for instance, that it is not difficult to uniquely distinguish individuals within these large data sets [8] because each of us has distinguishing features to our particular patterns of behavior. Several other papers have demonstrated that portions of these large data sets can be deanonymized, by matching these unique signatures across data sets. For such data sets, the only way to preserve privacy might be to not make the data available. Additionally, many of these really big data sets are collected by private companies, as the byproduct of their business, at a scale that scientists cannot achieve independently. These companies generally only provide access to the data if the data is not shared publicly, because they consider the data to be theirs [9]. If PLOS’s policy were universal, such data sets would seem to become inaccessible to science, and human knowledge would be unable to advance along any lines that require such data [10]. That does not seem like a good outcome.
PLOS does seem to acknowledge this issue, but in a very weak way, saying that “established guidelines” should be followed and privacy should be protected. For proprietary data sets, PLOS only makes this vague statement: “If license agreements apply, authors should note the process necessary for other researchers to obtain a license.” At face value, it would seem to imply that proprietary data sets are allowed, so long as other researchers are free to try to license them themselves, but the devil will be in the details of whether PLOS accepts such instructions or demands additional action as a requirement for publication. I’m not sure what to expect there.
On balance, I like and am excited about PLOS’s new data availability policy. It will certainly add some overhead to finalizing a paper for submission, but it will also make it easier to get data from previously published papers. And, I do think that PLOS put some thought into many of the issues identified above. I also sincerely hope they understand that some flexibility will go a long way in dealing with the practical issues of trying to achieve the ideal of open science, at least until we the community figure out the best way to handle these practical issues.
-----
[1] PLOS's Data Access for the Open Access Literature policy goes into effect 1 March 2014.
[2] See “The availability of Research Data Declines Rapidly with Article Age” by Vines et al. Cell 24(1), 94-97 (2013).
[3] Which, if they are published at a regular “restricted” access journal, they are not.
[4] For instance, there is a popular version of the Zachary Karate Club network that has an error, a single edge is missing, relative to the original paper. Fortunately, no one makes strong claims using this data set, so the error is not terrible, but I wonder how many people in network science know which version of the data set they use.
[5] There are some conditions for self-correction: there must be enough people thinking about a claim that someone might question its accuracy, one of these people must care enough to try to identify the error, and that person must also care enough to correct it, publicly. These circumstances are most common in big and highly competitive fields. Less so in niche fields or areas where only a few experts work.
[6] If you had a profile on Friendster or Myspace, do you know where your data is now?
[7] Federal law already prohibits sharing such sensitive information about human participants in research, and that law surely trumps any policy PLOS might want to put in place. I also expect that PLOS does not mean their policy to encourage the sharing of that sensitive information. That being said, their policy is not clear on what they would want shared in such cases.
[8] And, thus perhaps easier, although not easy, to identify specific individuals.
[9] And the courts seem to agree, with recent rulings deciding that a “database” can be copyrighted.
[10] It is a fair question as to whether alternative approaches to the same questions could be achieved without the proprietary data.
posted January 29, 2014 04:21 AM in Scientifically Speaking | permalink | Comments (3)
November 22, 2013
Network Analysis and Modeling (CSCI 5352)
This semester I developed and taught a new graduate-level course on network science, titled Network Analysis and Modeling (listed as CSCI 5352 here at Colorado). As usual, I lectured from my own set of notes, which total more than 150 pages of material. The class was aimed at graduate students, so the pace was relatively fast and I assumed they could write code, understood basic college-level math, and knew basic graph algorithms. I did not assume they had any familiarity with networks.
To some degree, the course followed Mark Newman's excellent textbook Networks: An Introduction, but I took a more data-centric perspective and covered a number of additional topics. A complete listing of the lecture notes are below, with links to the PDFs.
I also developed six problem sets, which, happily, the students tell me where challenging. Many of the problems were also drawn from Newman's textbook, although often with tweaks to make the more suitable for this particular class. It was a fun class to teach, and overall I'm happy with how it went. The students were enthusiastic throughout the semester and engaged well with the material. I'm looking forward to teaching it again next year, and using that time to work out some of the kinks and add several important topics I didn't cover this time.
--
Lecture 1,2 : An introduction and overview, including representing network data, terminology, types of networks (pdf)
Lecture 3 : Structurally "important" vertices, degree-based centralities, including several flavors of eigenvector centrality (pdf)
Lecture 4 : Geometric centralities, including closeness, harmonic and betweenness centrality (pdf)
Lecture 5 : Assortative mixing of vertex attributes, transitivity and clustering coefficients, and reciprocity (pdf)
Lecture 6 : Degree distributions, and power-law distributions in particular (pdf)
Lecture 7 : Degree distributions, and fitting models, like the power law, to data via maximum likelihood (pdf)
Lecture 8 : How social networks differ from biological and technological networks, small worlds and short paths (pdf)
Lecture 9 : Navigability in networks (discoverable short paths) and link-length distributions (pdf)
Lecture 10 : Probabilistic models of networks and the Erdos-Renyi random graph model in particular (pdf)
Lecture 11 : Random graphs with arbitrary degree distributions (the configuration model), their properties and construction (pdf)
Lecture 12 : Configuration model properties and its use as a null model in network analysis (pdf)
Lecture 13 : The preferential attachment mechanism in growing networks and Price's model of citation networks (pdf)
Lecture 14 : Vertex copying models (e.g., for biological networks) and their relation to Price's model (pdf)
Lecture 15 : Large-scale patterns in networks, community structure, and modularity maximization to find them (pdf)
Lecture 16 : Generative models of networks and the stochastic block model in particular (pdf)
Lecture 17 : Network inference and fitting the stochastic block model to data (pdf)
Lecture 18 : Using Markov chain Monte Carlo (MCMC) in network inference, and sampling models versus optimizing them (pdf)
Lecture 19 : Hierarchical block models, generating structurally similar networks, and predicting missing links (pdf)
Lecture 20 : Adding time to network analysis, illustrated via a dynamic proximity network (pdf)
--
Problem set 1 : Checking the basics and a few centrality calculations (pdf)
Problem set 2 : Assortativity, degree distributions, more centralities (pdf)
Problem set 3 : Properties and variations of random graphs, and a problem with thresholds (pdf)
Problem set 4 : Using the configuration model, and edge-swapping for randomization (pdf)
Problem set 5 : Growing networks through preferential or uniform attachment, and maximizing modularity (pdf)
Problem set 6 : Free and constrained inference with the stochastic block model (pdf)
posted November 22, 2013 11:00 PM in Teaching | permalink | Comments (1)
September 07, 2012
BioFrontiers is hiring
And, in more hiring news, the BioFrontiers Institute here at the University of Colorado Boulder is hiring new tenure-track faculty this year. One of the great things about this hiring line is that the candidate basically gets to select which of nine departments (including Computer Science, Physics, Applied Math., and a variety of biology departments) they want to call home. Another is that there are genuinely interesting and exciting things happening in research and education within it. In short: it's a great place to work.
We invite applications for a tenure-track faculty position from candidates seeking to develop an innovative research program that addresses significant problems in biology or medicine at the interface with the physical sciences, mathematics, and/or computer science. Researchers in the area of biological engineering are asked to look for an advertisement next year, when we anticipate searching for faculty in that area.
posted September 7, 2012 01:11 PM in Simply Academic | permalink | Comments (0)
September 06, 2012
Be an Omidyar Fellow at the Santa Fe Institute
The Santa Fe Institute is hiring new Omidyar Fellows.
Having spent four years at SFI as an Omidyar Fellow before coming to the University of Colorado, Boulder, I feel comfortable asserting that this fellowship is one of the best in higher education: it provides several years of full funding to work on your own big ideas about complex systems and to collaborate with other SFI researchers.
I also feel comfortable saying that SFI tends to prefer young scholars who are already fairly independent, as being an Omidyar Fellow is a lot like being a junior faculty member, except without the teaching responsibilities. No one tells you what to work on. If you have your own interests and your own ideas, this is ideal. SFI also tends to prefer young scholars with a strong quantitative background. If you plan to apply, I recommend writing a fresh research statement (rather than recycling the one you send to university positions) that focuses on your ideas about complex systems and your independent research plans for the next few years.
To help explain just how great a position it is, SFI recently put together a series of short videos, which you can find here.
Deadline: November 1
Apply online for the Omidyar Fellowship.
posted September 6, 2012 07:53 AM in Complex Systems | permalink | Comments (0)
May 21, 2012
If it disagrees with experiment, it is wrong
The eloquent Feynman on the essential nature of science. And, he nails it exactly: science is a process of making certain types of guesses about the world around us (what we call "theories" or hypotheses), deriving their consequences (what we call "predictions") and then comparing those consequences with experiment (what we call "validation" or "testing").
Although he doesn't elaborate them, the two transitions around the middle step are, I think, quite crucial.
First, how do we derive the consequences of our theories? It depends, in fact, on what kind of theory it is. Classically, these were mathematical equations and deriving the consequences meant deriving mathematical structures from them, in the form of relationships between variables. Today, with the rise of computational science, theories can be algorithmic and stochastic in nature, and this makes the derivation of their consequences trickier. The degree to which we can derive clean consequences from our theory is a measure of how well we have done in specifying our guess, but not a measure of how likely our guess is to be true. If a theory makes no measurable predictions, i.e., if there are no consequences that can be compared with experiment or if there is no experiment that could disagree with the theory, then it is not a scientific theory. Science is the process of learning about the world around us and measuring our mistakes relative to our expectations is how we learn. Thus, a theory that can make no mistakes teaches us nothing. [1]
Second, how do we compare our predictions with experiment? Here, the answer is clear: using statistics. This part remains true regardless of what kind of theory we have. If the theory predicts that two variables should be related in a certain way, when we measure those variables, we must decide to what degree the data support that relation. This is a subtle point even for experienced scientists because it requires making specific but defensible choices about what constitutes a genuine deviation from the target pattern and how large a deviation is allowed before the theory is discarded (or must be modified) [2]. Choosing an optimistic threshold is what gets many papers into trouble.
For experimental science, designing a better experiment can make it easier to compare predictions with data [3], although complicated experiments necessarily require sensitive comparisons, i.e., statistics. For observational science (which includes astronomy, paleontology, as well as many social and biological questions), we are often stuck with the data we can get rather than the data we want, and here careful statistics is the only path forward. The difficulty is knowing just how small or large a deviation is allowed by your theory. Here again, Feynman has something to say about what is required to be a good scientist:
I'm talking about a specific, extra type of integrity that is not lying, but bending over backwards to show how you are maybe wrong, that you ought to have when acting as a scientist. And this is our responsibility as scientists...
This is a very high standard to meet. But, that is the point. Most people, scientists included, find it difficult to be proven wrong, and Feynman is advocating the active self-infliction of these psychic wounds. I've heard a number of (sometimes quite eminent) scientists argue that it is not their responsibility to disprove their theories, only to show that their theory is plausible. Other people can repeat the experiments and check if it holds under other conditions. At its extreme, this is a very cynical perspective, because it can take a very long time for the self-corrective nature of science to get around to disproving celebrated but ultimately false ideas.
The problem, I think, is that externalizing the validation step in science, i.e., lowering the bar of what qualifies as a "plausible" claim, assumes that other scientists will actually check the work. But that's not really the case since almost all the glory and priority goes to the proposer not the tester. There's little incentive to close that loop. Further, we teach the next generation of scientists to hold themselves to a lower standard of evidence, and this almost surely limits the forward progress of science. The solution is to strive for that high standard of truth, to torture our pet theories until the false ones confess and we are left with the good ideas [4].
-----
[1] Duty obliges me to recommend Mayo's classic book "Error and the Growth of Experimental Knowledge." If you read one book on the role of statistics in science, read this one.
[2] A good historical example of precisely this problem is Millikan's efforts to measure the charge on an electron. The most insightful analysis I know of is the second chapter of Goodstein's "Fact or Fraud". The key point is that Millikan had a very specific and scientifically grounded notion of what constituted a real deviation from his theory, and he used this notion to guide his data collection efforts. Fundamentally, the "controversy" over his results is about this specific question.
[3] I imagine Lord Rutherford would not be pleased with his disciples in high energy physics.
[4] There's a soft middle ground here as to how much should be done by the investigator and how much should be done by others. Fights with referees during the peer-review process often seem to come down to a disagreement about how much and what kind of torture methods should be used before publication. This kind of adversarial relationship with referees is also a problem, I think, as it encourages authors to do what is conventional rather than what is right, and it encourages referees to nitpick or to move the goal posts.
posted May 21, 2012 04:29 PM in Scientifically Speaking | permalink | Comments (1)
February 22, 2012
Reinventing academia
Attention conservation notice: This post is about a talk in the Denver/Boulder area.
Jon Wilkins, a long-time colleague of mine at the Santa Fe Institute, and all around great guy, will be giving a talk at the Colorado School of Mines next week about his experience and efforts to create and maintain an independent scholarly institute, one that provides a virtual home for researchers unaffiliated with any existing research or higher education institution. The Ronin Institute is a true Internet-era research institute, having no physical location, only an electronic one.
"The Ronin Institute, or how to reinvent academia"
by Dr. Jon Wilkins, Ronin Institute
4:30 P.M., Tuesday, February 28, 2012
Alderson Hall Room 151
Abstract: After more than 10 years of working in traditional research institutions (Harvard University and the Santa Fe Institute), Dr. Wilkins founded the Ronin Institute with the objective to create an organization that can help to connect and support scholars who, by choice or by chance, do not have an affiliation with a university or other research institutes. In this lecture, Dr. Wilkins will share his motivation to found the institute, his long term vision, and how the Ronin Institute fits in the current academic ecosystem.
About the Speaker: Dr. Wilkins is an external professor at the Santa Fe institute and founder of the Ronin Institute. He received an A.B. degree in Physics from Harvard College in 1993, an M.S. degree in Biochemistry from the University of Wisconsin in 1998 and a Ph.D. in Biophysics from Harvard University in 2002. His interests are in evolutionary theory, broadly defined. His prior work has focused on coalescent theory and genomic imprinting. His current research has continued in those areas, and has expanded into areas like human language and demographic history, altruism, cultural evolution, and statistical inference.
posted February 22, 2012 08:14 AM in Simply Academic | permalink | Comments (2)
January 27, 2012
The cost of knowledge
Did you know that Congress is considering prohibiting the free dissemination of knowledge produced by federal research dollars? That's what the Research Works Act would do. The bill is backed by companies like Elsevier, who profit mainly from the volunteer labor of scientists (who produce the research and who vet the research for quality), and thus have a vested interest in preventing the free exchange of knowledge [1,2], or at least in extracting rents from everyone involved in it.
Other commercial publishers may not be as bad as Elsevier, but there are serious problems with them as well. Computers have arguably reduced the valued added by commercial publishers because they allow scientists to do themselves many of the tasks that publishers used to perform (like typesetting, spell checking, etc.), and they have virtually eliminated the cost of distribution and storage. Prof. Michael Eisen, writing in the New York Times [3], laid out the case for why open access publishing is not only realistic, but also morally responsible. To be honest, I am deeply sympathetic with these arguments and am reminded of them whenever I try to access journals when I'm off campus.
More recently, the Fields Medalist Tim Gowers [4] has started a petition to let working scientists declare their opposition to the Research Works Act [5] by promising to boycott Elsevier. (See also his explanation of why he's doing this.)
You can help by declaring that you (1) won't publish with them, (2) wont' referee for them, and/or (3) won't do editorial work for them. Please consider signing up, and also encouraging your colleagues to do the same:
Finally, John Baez has some thoughtful analysis of the problem, its origin and some potential solutions on his blog.
Tip to Slashdot.
Update 27 February: Elsevier has dropped support for the Research Works Act, and has written a letter to the mathematics community. The claim they will now reduce the overall cost they impose on the mathematics community, but in fact, this is merely a cynical sop because mathematics is a tiny part of Elsevier's portfolio.
-----
[1] Elsevier used to make some money from the military arms trade, but partly due to a furor raised by scientists, it eventually cut its ties to the international arms fairs in 2008. Given Elsevier's history, it seems unlikely that they would have made this choice without the public pressure the furor generated.
[2] Elsevier is perhaps the worst offender in the private scientific publishing industry. Their journals (even the crappy ones) typically cost significantly more than other private or non-profit publishers, they've even been caught taking money from the pharmaceutical industry in exchange for creating fake medical journals in which to publish fake research, and a few of their journals have been implicated in more academic types of fraud.
[3] Michael Eisen is one of the founders of the highly regarded Public Library of Science (PLoS), an open-access scientific publishing group. PLoS's founding story is relevant: it is well respected in the scientific community because many of its original journals were started by the members of journal editorial boards for Elsevier, who resigned en masse in protest over Elsevier's odious practices.
[4] The Fields Medal is a bit like a Nobel Prize for Mathematics.
[5] The bill's name really is a lovely example of Orwellian double speak.
posted January 27, 2012 07:02 AM in Simply Academic | permalink | Comments (3)
January 13, 2012
A crisis in higher education?
Attention conservation notice: 3200 cranky words on the PhD over-supply "crisis."
Higher education is always having crises, it seems. Some of this is probably a kind of marketing strategy, because genuinely serious problems are so systemic and slow-moving that it's easy to ignore them, or because you can't get people to pay attention in today's saturated media environment without a large dose of hyperbole. But, one "crisis" in particular did catch my attention over the past few years: the harsh market faced by relatively new PhDs seeking faculty jobs [0]. Nature did a full spread on the future of the PhD, The Economist weighed in with their own coverage, and I'm sure the Chronicle of Higher Education has done a number of stories on the topic. Now, Online PhD has done its own report, in the form of a slick infographic packed with grim factoids and numbers.
What most of these perspectives miss, and what makes some of their analysis a little shrill, is the historical context of higher education and its growth trajectory over the past 70 years [1]. The overall upward trend in PhD production over this time period can be blamed on huge increases in federal funding for research, on huge growth in the number of students getting undergrad degrees, on a vast broadening of higher education as a whole and on intensified competition between research-oriented universities.
The role of competition, I think, is under appreciated: many more universities now produce PhDs and many more programs are genuinely good than was the case before federal funding for higher education began surging after World War II. The result is a larger and more competitive market for those PhDs, especially the ones produced by the best programs. (The same is true for funding sources: the pie has grown, but the number of people competing for a slice has grown much faster.) In many ways, this is a good thing for higher education overall, since students can receive a good or even a great education at a wider variety of places. That is, higher education is probably genuinely less elitist and genuinely more accessible and inclusive. There's also a cost, however, in brutal treatment that even successful candidates experience on the market and in the colossal waste of human potential from the many talented individuals who fail to find good jobs. (More on "good" jobs in a moment.)
That being said, increased production of PhDs doesn't necessarily mean increased competition. If the number of tenure-track faculty positions increases at the same rate as the production of PhDs, then in principle competition could remain flat. This point gets a lot of attention in the popular discussion and the argument is often that if only we could increase the number of tenure-track lines, everything would be great. But this obscures the complexity of the problem. First, faculty lines are largely paid for by undergraduate (or professional degree) tuition [2], so increasing the size of the faculty requires increasing the size of the undergraduate population, which has its own problems. [3] Second, part of the modern National Science Foundation's mandate is actually to overproduce graduate students [4], and this is largely at the behest of Congress [5]. Alternatively, we could solve the over-supply of PhDs by reducing the overall production, but this would negatively impact the amount of research being produced (since it's largely done by PhD students), the teaching of many departments (again, often done by PhD students) and would reduce the supply of highly educated individuals to non-academic professions.
Third, not all tenure-track positions are equally desirable, not all PhDs are equally competitive, and growth in the most desirable slots and most competitive people has not been uniform. This is a tricky problem to explain but let's put it this way: I would not be surprised to learn that the 10 best programs in the country (in any particular field) collectively produce enough PhDs each year to fill every advertised faculty line at every other university, even the not-so-great ones [6]. This means that the lack-of-tenure-track-jobs / overproduction-of-PhDs "crisis" is not one that everyone feels equally, which complicates the conclusion that it is universally a problem. In fact, a tight job market for faculty positions has some benefits, at least collectively. One is that lower-quality places can pick up relatively better qualified people than they would if the top-ranked departments had enough extra lines to hire all the top applicants. Over time, an over-supply of good PhDs may be necessary to raise the quality of the worst-performing institutions, although this effect may only be observable in the long run. [7]
Fourth, the history of higher education as an industry is a series of large expansions and contractions, and the effects of these are often felt and distributed unevenly [8]. Life and job prospects for faculty in expanding fields are good, but are hard during contractions. (These effects are surely amplified for young scholars and so one possibility would be better knowing and advertising the true employment prospects for graduates; but maybe not [9].) It's not entirely clear to me that academia is actually experiencing a contraction, despite the federal budget travails. A more truthful statement may be that higher education is restructuring, which brings us to the issue of "good" jobs versus "bad" jobs.
It's true that universities (at least in the US) are increasingly made up of two types of faculty, those either with or who are eligible for tenure ("tenure track"; a population that is, at best, growing fairly slowly) and those without or who can never receive tenure (teaching faculty, adjuncts, etc.). The latter group is much larger now than it used to be, but it's not very well integrated into the decision-making systems of universities, and this, I think, leads to some level of systemic abuse. In the long run, it seems likely that these groups will become better integrated into the decision-make system, which will reduce the abuse [10]. But a more interesting question, I think, is why has this population grown so much so recently?
The role that universities play in society is changing, and I think the growth of these lower-quality jobs reflects this shift. The US economy overall has shifted significantly toward service-sector jobs and the growth in adjunct and teaching positions at universities should probably be seen as the higher education equivalent. This may be driven in part by the commoditization of a bachelors degree (which is primarily what non-tenure-track faculty help produce), which society has demanded and the universities have embraced (especially the big public universities and the non-endowed private colleges, where increased enrollment means increased tuition revenue). For their part, colleges and universities are figuring out that they can produce an apparently equivalent "product" at significantly lower cost by relying more heavily on non-tenure track faculty [11,12]. It seems telling that losses of tenure-track lines are often at colleges and universities well below the "top tier", where the struggle for product differentiation and the negative consequences of price competition are likely stronger. So, it seems reasonable to expect growth in these "bad" jobs in places where the service rendered (education provided) is less specialized, e.g., entry- or lower-level undergraduate classes where the material is highly standardized and probably does not require the best of the best minds to teach.
Another aspect is that tenure is not just about protecting faculty from being fired for political reasons. Tenure also allows universities to fulfill their mission toward preserving knowledge because tenured faculty will be around for a long time, communicating their vast and detailed knowledge to the next generation. Eliminating tenure lines may basically mean that an institution is giving up some or all of its commitment to the knowledge preservation mission. This is surely a loss for society as a whole, but it does raise the interesting question about which institutions are best positioned to fulfill that mission -- it may be that the institutions who are giving up on it were not doing a very good job at it in the first place. The fact that tenure lines are mainly (but not always) being lost from the lower-ranked institutions suggests that the top places are largely still committed to this mission, even if they are retrenching to some degree (perhaps because of the shifting demands on bachelor degree production described above).
So, let's take stock. Is there a crisis? Not in the usual definition of the word, no. But, there are serious issues that we should consider, and these tap deep into both the mission and purpose of higher education and its relationship to society as a whole.
The good things for society about the current system are that the over-supply of PhDs produces a steady stream of highly educated people for other industries and government to use. The over-supply means that low-quality departments will tend to improve over time because they can hire better people than their peers tend to produce. The over-supply also means that the best or most desirable departments will also tend to improve over time because they can select their new hires from the very best of the very best. For scholarship in general, this is a good thing. The over-supply means that academia has a large supply of low-cost skilled labor (graduate students) for producing research, educating younger students, etc. And, the over-supply means that academia has an adequate supply of potential faculty to facilitate restructuring needs, i.e., responding to the changing demands from society and the changing roles of universities.
The bad things are that the over-supply is a colossal waste of human potential for people who aspire to be faculty but who ultimately fail to find employment. For instance, many very talented individuals will spend substantial time in low-paying, low-benefits temporary employment (graduate students, postdocs, adjuncts, research faculty positions, etc.) only to discover years or decades later that these years are now counted against them on the job market (and not just in the academic market). The over-supply makes the individual experience of finding a job fairly brutal and with a high error rate (many people who should get faculty jobs do not [13]). Success also comes with a cost in the form of moving a very large distance (the faculty job market is one of the few truly national labor markets). The over-supply has made it easy for susceptible colleges and universities to slowly replace their tenure track faculty with non-tenure faculty with less autonomy, less security, lower pay and lower benefits, which ultimately means these institutions basically abandon one of their missions: preserving human knowledge. It also makes the institution less democratic, which likely has a negative impact on the campus culture and the educational environment.
Just as this situation did not appear suddenly, I don't think it will change significantly in the near future. Although Congress is a powerful voice in higher education, and has had a direct role in creating the over-supply, the large and complex ecology of higher education institutions, society itself and the economy as a whole are also key players. What happens will depend on their interactions, and lobbying Congress alone may lead to unexpected and undesirable results [14]. In the near term, I think the over-supply will persist (and if anything the job market will become even more competitive, but again this is not a completely bad thing), the number of non-tenured positions will continue to increase (mainly at lower-ranked institutions or for teaching intro classes at the higher-ranked places), undergraduate degrees will become even more comoditized, and the mission of knowledge preservation will be increasingly concentrated among the better or more financially stable institutions.
One long-term consequence is a partitioning of the faculty at research universities into "research" faculty (tenure-track faculty who do research and teach mainly graduate and upper-level undergraduate courses, of which I am one) and "teaching" faculty (non-tenure track faculty who teach heavy course loads of lower-level undergraduate classes), but that does seem like the way things are going [15]. I wish that research universities (and tenure-track faculty) would treat the non-tenure groups with more respect and include them more directly into the decision-making processes. And, I hope that we can find better ways of encouraging the very best young scholars to stick with it, even though the system will likely become only more brutal in the future [16].
To end on a more positive note, one genuinely beneficial thing we as academics could do would be to encourage our PhD students to consider non-academic trajectories. That is, I don't think we should view the PhD as being exclusively an academic degree, and we could strive to teach our PhD students a combination of both academic and practical skills. This would increase their options on the job market, which may reduce the overall brutality that individuals currently experience.
-----
[0] Partly because I was in that market myself. And, now being in a tenure-track position at a good university, I'm lucky enough to be on the other side of that harrowing process. Had I written this a couple of years ago, I'm sure I would have said slightly different things.
[1] These are well covered by Roger Geiger's excellent and authoritative books on the evolution of the American university system, in the post-war period and since 1990. These books are highly recommended. Geiger takes what should be a dry and boring subject and makes it a fascinating and insightful story.
[2] This is true at both public and private universities. The only place it's less accurate is in medical research schools, where faculty lines are often funded out of "soft" money from research grants. (Some are still funded from medical school tuition revenue, so the coupling with student populations is not eliminated.) The result is that these faculty lines are mainly sensitive to changes in federal funding levels.
[3] Another complicating factor is that tenure lines are traditionally tied to departments, and their number depends on student demand for those courses offered by that department. That is, teaching is still a labor-constrained activity. The division of that labor into departments means that growth in faculty lines is driven by changes in the popularity of different disciplines. The benefits for the faculty job market created by overall growth in student enrollments will thus be distributed unevenly.
There are at least two factors that decouple the number of faculty lines and the popularity of the field: tenure, which means departments tend to shrink very slowly in response to decreasing popularity while the reverse is not true, and the commitment that all universities have to the preservation and production of knowledge, which means even an unpopular department may be maintained as a kind of cultural memory device.
[4] This is done partly through direct support to students (about 15% of the budget) and partly through grants (50% of the budget); typically, large portions of grants are in fact used to support graduate students by paying them as research assistants.
[5] Apparently, NSF has always struggled to justify its budget to Congress, who generally has an uncomfortable relationship with the idea of supporting basic research for the sake of humanity. For NSF, historically "supporting education," and more recently "supporting economic growth" (a.k.a. technology transfer), have been a successful arguments, and these are reflected in funding priorities.
[6] This is almost surely true in Computer Science, where some of the best programs are also some of the largest. For example, MIT and CMU collectively have about 250 faculty; if they each produce a single graduate each year, that would be enough to place one person at each of the other 200-odd PhD-granting Computer Science departments in North America. The per-faculty production rate is probably not so high, the overall volume may be so if we account for other top places like Stanford, Washington, Princeton, etc. If we include the fact that not every department hires every year, it seems entirely reasonable that the top 10 places could fill the entire annual market demand themselves.
[7] This effect probably happens faster for newer fields, e.g., complex systems. The best universities are all fairly sensitive to their perceived prestige and quality, and for them, it doesn't make strategic sense to risk their current standing with risky investments in untested fields. This means that lower-ranked universities who place smart bets can move up (at least during the time it takes for a field to become established enough that the top places start poaching the best people).
[8] Physics experienced a long expansion, but that had largely run its course in the United States by the time Congress trashed the Superconducting Super Collider in 1993. In contrast, biomedical research has been expanding fairly steadily for 70 years, which is probably why it dominates federal science budgets. The "golden age" of science was really the post-war and Sputnik eras, when federal spending was expanding faster than universities could satisfy the demand for research. The 1970s were apparently a fairly broad contraction, because Congress moved to limit the growth in science budgets (for instance, NASA's budget peaked in the early 1970s) and because student enrollment growth tempered. Since then, the expansions and contractions have been less even.
[9] On the other hand, had anyone convincingly explained to my younger self just what life would be like in my first few years as a professor, I may have decided to try a different career path. More generally, ignorance may be a crucial part of what makes the whole system work: it allows us to unknowingly take foolish risks that sometimes yield truly remarkable, or at least highly improbable, results. At the collective level, youthful foolishness may be essential to keeping the quality of the faculty high despite the brutality of the career path.
[10] Of course, in the meantime it's terrible that some institutions and individuals are taking advantage of the current powerlessness of these groups. They can and should be integrated into the academy and given a voice.
[11] Some graduate and professional degrees also show evidence of becoming commodities, for instance, MBAs. It's not clear that PhDs are facing similar pressures, although in my darker moments I believe it.
[12] From this perspective, things like Stanford's free online courses may be a truly disruptive innovation. They offer the possibility of dramatically lowered cost, dramatically increased "production" and they seem to require a currently specialized set of skills. Of course, their success could destroy what remains of the tenure track at smaller or less unique institutions.
[13] As I've learned, it's a highly stochastic and error-prone process. Departments tend to decide ahead of time to hire in a particular area, and this means top-notch candidates from outside that area are potentially passed over for less-top-notch candidates within the target area. The decision of which area to hire in is often driven by internal politics (which "group" is stronger, which has a louder voice, "who's turn" it is) or existing curricular needs rather than meritocratic or strategic concerns. And, even within a given area, it can be difficult to accurately access true merit and relative quality, particularly for junior positions where each candidate's track record is, by definition, relatively short.
Did I mention that I'm reading PhD applications this week? Ugh.
[14] It certainly has in the past.
[15] Ironically, dividing the teaching and research between different groups of faculty is mostly how the Germans used to do things. Now, the Germans are Americanizing their system to some degree, while we Americans seem to be Germanizing ours.
[16] From my perspective, "early career" funding, fellowship and other young faculty support mechanisms seem to be wholly inadequate (in size and scope) and the easiest way to get them is to propose highly incremental, highly risk-averse research. This does not seem to be serving the right goals or to be teaching young faculty the right lessons about scholarship.
posted January 13, 2012 10:26 AM in Simply Academic | permalink | Comments (8)
March 25, 2011
Why scientists are different
xkcd on why scientists are different from non-scientists.
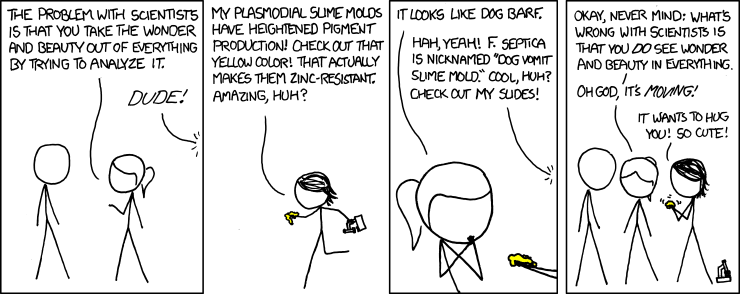
posted March 25, 2011 10:01 AM in Humor | permalink | Comments (1)
November 04, 2010
Peer review and the meat grinder
ArsTechnica's Chris Lee has a nice (and brief) meditation on peer review, and it's suitability for vetting both research papers and grant proposals. The title gives away a lot "A trip through the peer review sausage grinder", but that should be no surprise to anyone who lives with the peer-review process. The punch line Lee comes to is that peer review works okay at vetting the results of scientific research but fails at vetting potential research, that is, grant proposals. This conclusion seems entirely reasonable to me. [1]
Given some interactions with NSF Program Managers and related folks over the past year, peer review is the one thing that is not up for discussion at NSF. [2] They're happy to hear broad-based appeals for more funding, for suggestions about different types of funding, etc. But they are adamantly attached to sending grant proposals out for review by other scientists and taking the advise they get back seriously. To be honest, I'm not sure how else they could do it. As Lee points out, there are many more scientists now than there is funding, and the fundamental question is how do we allocate money to the projects and people most likely to produce interesting and useful results? This kind of pre-judgement of ultimate quality is fundamentally hard; peer-review frequently fails at doing this for research that is already finished (peer review at journals), and is even worse for research that has not yet been done (peer review for grant proposals). Track-record-based systems are biased against young scholars; shrinking the size of the applicant pool smacks of elitism; and peer-review effectively produces boring, incremental research. Lee suggests a lottery-based system, which is an interesting idea, but it would never fly.
-----
[1] A part of me is proud of the fact that one of my proposals at NSF was criticized both for being too ambitious and for being too incremental.
[2] Here and here are NSF's explanations for why peer review is good and how it works.
posted November 4, 2010 07:55 AM in Simply Academic | permalink | Comments (0)
October 27, 2010
Story-telling, statistics, and other grave insults
The New York Times (and the NYT Magazine) has been running a series of pieces about math, science and society written by John Allen Paulos, a mathematics professor at Temple University and author of several popular books. His latest piece caught my eye because it's a topic close to my heart: stories vs. statistics. That is, when we seek to explain something [1], do we use statistics and quantitative arguments using mainly numbers or do we use stories and narratives featuring actors, motivations and conscious decisions? [2] Here are a few good excerpts from Paulos's latest piece:
...there is a tension between stories and statistics, and one under-appreciated contrast between them is simply the mindset with which we approach them. In listening to stories we tend to suspend disbelief in order to be entertained, whereas in evaluating statistics we generally have an opposite inclination to suspend belief in order not to be beguiled. A drily named distinction from formal statistics is relevant: we’re said to commit a Type I error when we observe something that is not really there and a Type II error when we fail to observe something that is there. There is no way to always avoid both types, and we have different error thresholds in different endeavors, but the type of error people feel more comfortable may be telling.
...
I’ll close with perhaps the most fundamental tension between stories and statistics. The focus of stories is on individual people rather than averages, on motives rather than movements, on point of view rather than the view from nowhere, context rather than raw data. Moreover, stories are open-ended and metaphorical rather than determinate and literal.
It seems to me that for science, the correct emphasis should be on the statistics. That is, we should be more worried about observing something that is not really there. But as humans, statistics is often too dry and too abstract for us to understand intuitively, to generate that comfortable internal feeling of understanding. Thus, our peers often demand that we give not only the statistical explanation but also a narrative one. Sometimes, this can be tricky because the structure of the two modes of explanation are in fundamental opposition, for instance, if the narrative must include notions of randomness or stochasticity. In such a case, there is no reason for any particular outcome, only reasons for ensembles or patterns of outcomes. The idea that things can happen for no reason is highly counter intuitive [3], and yet in the statistical sciences (which is today essentially all sciences), this is often a critical part of the correct explanation [4]. For the social sciences, I think this is an especially difficult balance to strike because our intuition about how the world works is built up from our own individual-level experiences, while many of the phenomena we care about are patterns above that level, at the group or population levels [5].
This is not a new observation and it is not a tension exclusive to the social sciences. For instance, here is Stephen J. Gould (1941-2002), the eminent American paleontologist, speaking about the differences between microevolution and macroevolution (excerpted from Ken McNamara's "Evolutionary Trends"):
In Flatland, E.A. Abbot's (1884) classic science-fiction fable about realms of perception, a sphere from the world of three dimensions enters the plane of two-dimensional Flatland (where it is perceived as an expanding circle). In a notable scene, he lifts a Flatlander out of his own world and into the third dimension. Imagine the conceptual reorientation demanded by such an utterly new and higher-order view. I do not suggest that the move from organism to species could be nearly so radical, or so enlightening, but I do fear that we have missed much by over reliance on familiar surroundings.
An instructive analogy might be made, in conclusion, to our successful descent into the world of genes, with resulting insight about the importance of neutralism in evolutionary change. We are organisms and tend to see the world of selection and adaptation as expressed in the good design of wings, legs, and brains. But randomness may predominate in the world of genes--and we might interpret the universe very differently if our primary vantage point resided at this lower level. We might then see a world of largely independent items, drifting in and out by the luck of the draw--but with little islands dotted about here and there, where selection reins in tempo and embryology ties things together. What, then, is the different order of a world still larger than ourselves? If we missed the world of genic neutrality because we are too big, then what are we not seeing because we are too small? We are like genes in some larger world of change among species in the vastness of geological time. What are we missing in trying to read this world by the inappropriate scale of our small bodies and minuscule lifetimes?
To quote Howard T. Odum (1924-2002), the eminent American ecologist, on a similar theme: "To see these patterns which are bigger than ourselves, let us take a special view through the macroscope." Statistical explanations, and the weird and diffuse notions of causality that come with them, seem especially well suited to express in a comprehensible form what we see through this "macroscope" (and often what we see through microscopes). And increasingly, our understanding of many important phenomena, be they social network dynamics, terrorism and war, sustainability, macroeconomics, ecosystems, the world of microbes and viruses or cures for complex diseases like cancer, depend on us seeing clearly through some kind of macroscope to understand the statistical behavior of a population of potentially interacting elements.
Seeing clearly, however, depends on finding new and better ways to build our intuition about the general principles that take inherent randomness or contingency at the individual level and produce complex patterns and regularities at the macroscopic or population level. That is, to help us understand the many counter-intuitive statistical mechanisms that shape our complex world, we need better ways of connecting statistics with stories.
27 October 2010: This piece is also being featured on Nature's Soapbox Science blog.
-----
[1] Actually, even defining what we mean by "explain" is a devilishly tricky problem. Invariably, different fields of scientific research have (slightly) different definitions of what "explain" means. In some cases, a statistical explanation is sufficient, in others it must be deterministic, while in still others, even if it is derived using statistical tools, it must be rephrased in a narrative format in order to provide "intuition". I'm particularly intrigued by the difference between the way people in machine learning define a good model and the way people in the natural sciences define it. The difference appears, to my eye, to be different emphases on the importance of intuitiveness or "interpretability"; it's currently deemphasized in machine learning while the opposite is true in the natural sciences. Fortunately, a growing number of machine learners are interested in building interpretable models, and I expect great things for science to come out of this trend.
In some areas of quantitative science, "story telling" is a grave insult, leveled whenever a scientist veers too far from statistical modes of explanation ("science") toward narrative modes ("just so stories"). While sometimes a justified complaint, I think completely deemphasizing narratives can undermine scientific progress. Human intuition is currently our only way to generate truly novel ideas, hypotheses, models and principles. Until we can teach machines to generate truly novel scientific hypotheses from leaps of intuition, narratives, supported by appropriate quantitative evidence, will remain a crucial part of science.
[2] Another fascinating aspect of the interaction between these two modes of explanation is that one seems to be increasingly invading the other: narratives, at least in the media and other kinds of popular discourse, increasing ape the strong explanatory language of science. For instance, I wonder when Time Magazine started using formulaic titles for its issues like "How X happens and why it matters" and "How X affects Y", which dominate its covers today. There are a few individual writers who are amazingly good at this form of narrative, with Malcolm Gladwell being the one that leaps most readily to my mind. His writing is fundamentally in a narrative style, stories about individuals or groups or specific examples, but the language he uses is largely scientific, speaking in terms of general principles and notions of causality. I can also think of scientists who import narrative discourse into their scientific writing to great effect. Doing so well can make scientific writing less boring and less opaque, but if it becomes more important than the science itself, it can lead to "pathological science".
[3] Which is perhaps why the common belief that "everything happens for a reason" persists so strongly in popular culture.
[4] It cannot, of course, be the entire explanation. For instance, the notion among Creationists that natural selection is equivalent to "randomness" is completely false; randomness is a crucial component of way natural selection constructs complex structures (without the randomness, natural selection could not work) but the selection itself (what lives versus what dies) is highly non-random and that is what makes it such a powerful process.
What makes statistical explanations interesting is that many of the details are irrelevant, i.e., generated by randomness, but the general structure, the broad brush-strokes of the phenomena are crucially highly non-random. The chief difficulty of this mode of investigation is in correctly separating these two parts of some phenomena, and many arguments in the scientific literature can be understood as a disagreement about the particular separation being proposed. Some arguments, however, are more fundamental, being about the very notion that some phenomena are partly random rather than completely deterministic.
[5] Another source of tension on this question comes from our ambiguous understanding of the relationship between our perception and experience of free will and the observation of strong statistical regularities among groups or populations of individuals. This too is a very old question. It tormented Rev. Thomas Malthus (1766-1834), the great English demographer, in his efforts to understand how demographic statistics like birth rates could be so regular despite the highly contingent nature of any particular individual's life. Malthus's struggles later inspired Ludwig Boltzmann (1844-1906), the famous Austrian physicist, to use a statistical approach to model the behavior of gas particles in a box. (Boltzmann had previously been using a deterministic approach to model every particle individually, but found it too complicated.) This contributed to the birth of statistical physics, one of the three major branches of modern physics and arguably the branch most relevant to understanding the statistical behavior of populations of humans or genes.
posted October 27, 2010 07:15 AM in Scientifically Speaking | permalink | Comments (0)
August 22, 2010
Postdoc in Computational Biology at CU Denver
The Computational Biosciences Program at CU Denver is affiliated with the Colorado Initiative for Molecular Biotechnology that I'm involved with at CU Boulder. I got to interact with them when I interviewed at CU Boulder, and it seems like a very good program. All the better that it's co-located with CU's medical school, which is in Denver not Boulder. If you're into computational biology, this postdoctoral fellowship should be a great opportunity. I should also add that if you wanted to work with me at CU Boulder, I believe you could do that under this fellowship. The bad news is that the submission deadline is September 1st.
Postdoctoral Fellowships in Computational Bioscience
The Computational Bioscience Program at the University of Colorado, Denver School of Medicine is recruiting for three NLM (NIH) funded postdoctoral fellow. The Computational Bioscience Program is home to ten core faculty working in the areas of genomics, text mining, molecular evolution, phylogenetics, network analysis, statistical methods, microarray, biomedical ontology, and other areas. The School of Medicine is home to a broad array of outstanding research and instrumentation, including a 900 MHz NMR, extensive DNA sequencing and microarray facilities, and more. We are housed on the first all-new medical campus of the 21st century, close to both the urban amenities of Denver and the beautiful Rocky Mountains. For more information, please consult http://compbio.ucdenver.edu.
QUALIFICATIONS: Candidates must have a Ph.D. degree in Computational Biology or a related discipline, and be U.S. Citizens or Permanent Residents.
SALARY/BENEFITS: Successful candidates will be offered the NRSA specified stipend (based on years of experience), medical insurance, $2000 per year in travel support and $6500 per year in additional research-related expenses.
TO APPLY: Send to liz.pruett@ucdenver.edu a cover letter, curriculum vitae, and a statement of research interests; also arrange for three letters of recommendation to be sent to the same email address.
Priority will be given to applicants that apply by September 1, 2010.
UC Denver is an equal opportunity employer. Women and minorities are especially encouraged to apply.
posted August 22, 2010 06:59 AM in Simply Academic | permalink | Comments (1)
August 16, 2010
Phase change
Today I started work as an Assistant Professor of Computer Science at the University of Colorado at Boulder.
My three and a half years as a postdoc at the Santa Fe Institute were intense and highly educational. As I've been saying recently when people asked me, I feel like I really found my own voice as a young scholar at SFI, developing my own perspective on the general areas I work in, my own research agenda for the foreseeable future, and a distinct approach to scientific problems. I've also written a few papers that, apparently, a lot of people really like.
As a professor now, I get to learn a lot of new stuff including how to teach, how to build and run a research group, and how to help run a department, among other things. I hope this next phase is as much or even more fun than the last one. I plan to continue to blog as regularly as I can, and probably about many of the same topics as before, along with new topics I become interested in as a result of hanging out more with computer scientists. Should be fun!
posted August 16, 2010 09:46 AM in Self Referential | permalink | Comments (0)
July 21, 2010
Learning to say "no"
I'm not sure I learned this when I was in graduate school, but I'm definitely having to learn it now...
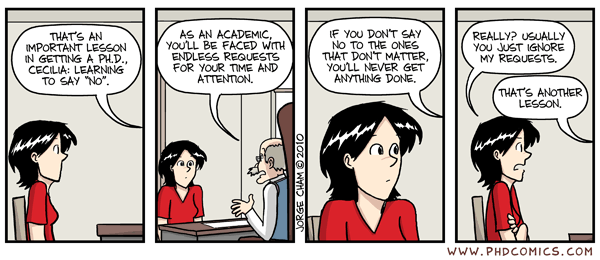
posted July 21, 2010 07:31 AM in Humor | permalink | Comments (2)
July 18, 2010
Academic job market
This image popped up as a joke in a talk I saw recently at Stanford, and it generated snide remarks like "3 postdocs and only 6 papers? No wonder he's desperate" and "He must be a physicist" [1].
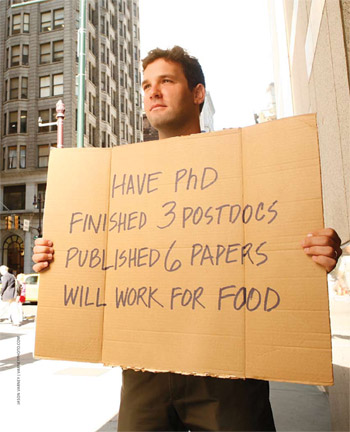
But, from the gossip I hear at conferences and workshops, the overall academic job market does seem to be this bad. Last year, I heard of universities canceling their faculty searches (sometimes after receiving submissions), and very well qualified candidates coming up empty handed after sending out dozens of applications [2]. I've heard much less noise this year (probably partly because I'm not on the job market), but everyone's expectation still seems to be that faculty hiring will remain relatively flat for this year and next. This seems sure to hurt young scholars the most, as there are only three ways to exit the purgatory of postdoctoral fellowships while continuing to do research: get a faculty job, switch into a non-tenure-track position ("staff researcher", "adjunct faculty", etc.), or quit academia.
Among all scientists, computer scientists may have the best options for returning to academia after spending time in industry (it's a frequent strategy, particularly among systems and machine learning people), followed perhaps by statisticians, since the alignment of academic research and industrial practice can be pretty high for them. Other folks, particularly theoreticians of most breeds, probably have a harder time with this leave-and-come-back strategy. The non-tenure-track options seem fraught with danger. At least, my elders have consistently warned me that only a very very small fraction of scientists return to active research in a tenure-track position after sullying their resumes with such a position [3].
The expectation among most academics seems to be that with fewer faculty jobs available, more postdocs may elect to stay in purgatory, which will increase the competition for the few faculty jobs that do exist over the next several years. The potential upside for universities is that lower-tier places will be able to recruit more highly qualified candidates than usual. But, I think there's a potential downside, too: some of the absolute best candidates may not wait around for decent opportunities to open up, and this may ultimately decrease the overall quality of the pool. I suppose we'll have to wait until the historians can sort things out after-the-fact before we know which, or how much of both, of these will actually happen. In the mean time, I've very thankful that I have a good faculty job to move into.
Update 20 July 2010: The New York Times today ran a series of six short perspective pieces on the institution of tenure (and the long and steady decline in the fraction of tenured professors). These seem to have been stimulated by a piece in the Chronicle of Higher Education on the "death" of tenure, which argues that only about a quarter of people teaching in higher education have some kind of tenure. It also argues that the fierce competition for tenure-track jobs discourages many very good scholars from pursuing an academic career. Such claims seems difficult to validate objectively, but they do seem to ring true in many ways.
-----
[1] In searching for the original image on the Web, I learned that it was apparently produced as part of an art photo shoot and the gent holding the sign is one Kevin Duffy, at the time a regional manager at the pharma giant Astra Zeneca and thus probably not in need of gainful employment.
[2] I also heard of highly qualified candidates landing good jobs at good places, so it wasn't doom and gloom for everyone.
[3] The fact that this is even an issue, I think, points to how pathologically narrow-minded academics can be in how we evaluate the "quality" of candidates. That is, we use all sorts of inaccurate proxies to estimate how "good" a candidate is, things like which journals they publish in, their citation counts, which school they went to, where they've worked, who wrote their letters of recommendation, whether they've stayed on the graduate school-postdoc-faculty job trajectory, etc. All of these are social indicators and thus they're merely indirect measures of how good a research a candidate actually is. The bad news is that they can, and often are, gamed and manipulated, making them not just noisy indicators but also potentially highly biased.
The real problem is twofold. First, there's simply not enough time to actually review the quality of every candidate's body of work. And second, science is so large and diverse that even if there were enough time, it's not clear that the people tasked with selecting the best candidate would be qualified to accurately judge the quality of each candidate's work. This latter problem is particularly nasty in the context of candidates who do interdisciplinary work.
posted July 18, 2010 10:13 AM in Simply Academic | permalink | Comments (2)
July 16, 2010
Confirmation bias in science
There's a decent meditation by Chris Lee on the problems of confirmation bias in science over at Nobel Intent, ArsTechnica's science corner. In its simplest form, confirmation bias is a particularly nasty mistake to make for anyone claiming to be a scientist. Lee gives a few particularly egregious (and famous) examples, and then describes one of his own experiences in science as an example of how self-corrective science works. I particularly liked the analogy he uses toward the end of the piece, where he argues that modern science is like a contact sport. Certainly, that's very much what the peer review and post-publication citation process can feel like.
Sometimes, however, it can take a long time for the good ideas to emerge out of the rough and tumble, particularly if the science involves complicated statistical analyses or experiments, if good data is hard to come by (or if the original data is unavailable), if there are strong social forces incentivizing the persistence of bad ideas (or at least, if there's little reward for scientists who want to sort out the good from the bad, for instance, if the only journals that will publish the corrections are obscure ones), or if the language of the field is particularly vague and ill-defined. [1]
Here's one of Lee's closing thoughts, which I think characterizes how science works when it is working well. The presence of this kind of culture is probably a good indicator of a healthy scientific community.
This is the difference between doing science from the inside and observing it from the outside. [Scientists] attack each other's ideas mercilessly, and those attacks are not ignored. Sometimes, it turns out that the objection was the result of a misunderstanding, and once the misunderstanding is cleared up, the objection goes away. Objections that are relevant result in ideas being discarded or modified. And the key to this is that the existence of confirmation bias is both acknowledged and actively fought against.
-----
[1] Does it even need to be said?
posted July 16, 2010 04:55 PM in Scientifically Speaking | permalink | Comments (0)
July 01, 2010
Life as a young scholar
A few months ago, I ran a mini-workshop with some of the other postdocs here at SFI [1] on getting into the habit of tracking your professional activities as a young scholar. My own experience, and my impression from talking with other young scientists, is that this is a delicate time in our careers (that is, the postdoc and early pre-tenure years) [2]. And, getting into the habit of keeping track of our professional lives is one way, I think, to help make all this work pay off down the road, for example, when we apply for faculty jobs or go up for tenure or otherwise need to show that we've been actually productive scientists. [3]
The basic point of the mini-workshop was for me to give them a template I've developed for tracking my own professional activities (here as tex and pdf). This helps me keep track of things like the papers I write and publish, talks I give, manuscripts I referee, interactions with the press, interactions with funding agencies, teaching and mentoring, "synergistic" activities like blogging, and the various opportunities I've declined. A side benefit for being mildly compulsive about this is that at the end of the year, when I'm questioning whether I've accomplished anything at all over the past year, I can look back and see just how much (or little) I did.
-----
[1] Incidentally, for those of you thinking of applying to SFI for the Omidyar Fellowship this year, be forewarned that the application deadline will almost surely be earlier this year than last year. It may be as early as mid-September.
[2] Delicate because many of us are no longer primarily publishing with our famous, or at least relatively well-known advisors. Just because a paper is good or even truly ground breaking doesn't mean it will be widely read, even among its primary audience. To be read, it needs to be noticed and recognized as being potentially valuable. Academics, being short on time and having only a local view of an ever-expanding literature, naturally resort to a variety of proxies for importance. Things like what journal it appeared in, whether they recognize any of the authors' names, how many citations it has, etc. A consequence is that many papers that are utter rubbish in fact are widely read and cited perhaps mainly because they scored highly on these proxies. For instance, they might have appeared in a vanity journal like Nature, Science or PNAS, or they might have had a famous person's name on them. (There are even some scientists who have made an entire career on gaming these kinds of proxies.) And, there's some evidence that this perception is not mere academic jealousy or sour grapes, but rather a measurable sociological effect.
The point here is that young scholars face a brutal competition to distinguish themselves and join the ranks of respected, recognized scientists. The upside of this struggle is learning more about how to get papers published, how to write for certain kinds of journals, how to play the grants game, and, hopefully, increased name recognition. Is it even controversial to argue that academia is a reputation-based system? The downside of this struggle is that many talented young scholars give up before gaining that recognition. [4]
[3] There are other tools out there for tracking your activities at a more fine-grained level (like Onlife for the Mac), but I don't use them. I tried one a while back, but found that it didn't really help me understand anything and was a mild distraction to getting real work done.
[4] If you'd like another explanation of why the process of becoming a respected scientist is so brutal, you might try the Miller-McCune cover story from few weeks ago titled "The Real Science Gap". The basic argument is that, contrary to what we hear in the media, there's a huge surplus of young scholars in the system. But, these budding scientists face a huge shortfall in opportunities for professional advancement, are faced with institutional mechanisms that underpay and undervalue them, and these cause most to drop out of science. The author seems to think a good solution would be to reduce the number of PhDs being produced, back to pre-WW2 levels, which would thus increase the likelihood that a newly minted PhD ends up with as a professor. But, this misses the point, I think, and would return science to its elitist roots. A better solution would be to give young scholars at all levels in science better pay, more opportunities for advancement that don't end in a tenure-track faculty job, and more respect for their contributions to science. And, critically, do a better job of explaining what the true academic job market is like.
posted July 1, 2010 04:40 PM in Simply Academic | permalink | Comments (0)
May 03, 2010
How to give a good (professional) talk
Partly because of my year-in-review blog posts (e.g., 2009) and partly because I actively keep track of this kind of stuff (to convince myself at the end of each year that I have actually gotten some things done over the past 12 months), I know that I've now given about 65 professional talks of various kinds over the past 7 years. I cringe to think about what my first few talks must have been like, and I cringe only a little less to think about my current talks. I'm sure that after another 650, I'll still be trying to figure out how to give better talks.
That being said, I do think I can recognize good advice when I see it (even if I have a hard time following it), and John E. McCarthy's advice [1] is pretty darn good. Some of it is specific to mathematics talks, but most of the points are entirely general. Here are the main bullet points (on each of which McCarthy further elaborates):
1. Don't be intimidated by the audience.
2. Don't try to impress the audience with your brilliance.
3. The first 20 minutes should be completely understandable to graduate students.
4. Carry everyone along.
5. Talk about examples.
8. Pay attention to the audience.
9. Don’t introduce too many ideas.
11. Find out in advance how long the colloquium is, and prepare accordingly.
13. You do not have to talk about your own work.
I also found Scott Berkun's book "Confessions of a Public Speaker" to be both entertaining and useful. Berkun is a professional public speaker who works the technology circuit, but a lot of his advice holds just as well for scientific talks. Here's a selection of his advice (some paraphrased, some quoted):
1. Take a strong position in your title.
2. Think carefully about your specific audience.
3. Make your specific points as concise as possible.
4. Know the likely counter arguments from an intelligent, expert audience.
5. You are the entertainment. Do your job.
6. A good talk builds up to a few simple take-home messages.
7. Know what happens next (in your talk).
8. The easiest way to be interesting is to be honest.
9. Do not start with your slides. Start by thinking about and understanding your audience.
10. Practicing your talk will make it much much better.
A couple of years ago, SFI hired a professional "talk" coach. One of her main suggestions, and one shared by Berkun, is that we should each video ourselves giving a talk and then watch it several times to see what exactly we do that we're not aware of. This is a cringe-worthy experience [2] but I can tell you it's highly useful. I think we as speakers are often completely unaware of our nervous tics or obnoxious speaker habits. Watching yourself on video is perhaps the only way to get unbiased feedback about them.
Tip to Cris.
-----
[1] A little Googling suggests that McCarthy's advice originally appeared in Canadian Mathematical Society NOTES in 1999 and was then reprinted by the American Mathematical Society. And, it seems to have a long history of being passed around since then.
[2] "OMG. Do I really sound like that?" and "Woah. Do I really do that when I talk?"
posted May 3, 2010 02:33 PM in Simply Academic | permalink | Comments (0)
April 12, 2010
As a real doctor...
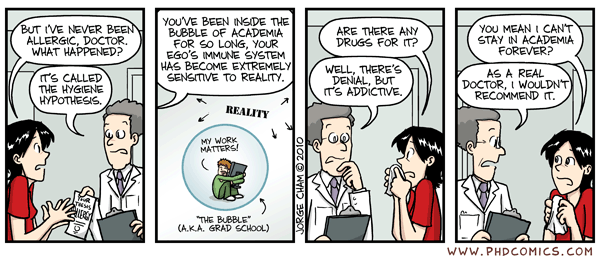
posted April 12, 2010 10:28 AM in Humor | permalink | Comments (0)
January 08, 2010
Facebook Fellowships 2010
These sound like a great opportunity for folks doing doctoral work on complex networks, and related topics. Facebook says they're only for this school year, but I suspect that if they get some good applications, and if the people who get the fellowships do good work, that they'll do this again next year.
Facebook Fellowships:
Every day Facebook confronts among the most complex technical problems and we believe that close relationships with the academy will enable us to address many of these problems at a fundamental level and solve them. As part of our ongoing commitment to academic relations, we are pleased to announce the creation of a Facebook Fellowship program to support graduate students in the 2010-2011 school year.
We are interested in a wide range of academic topics, including the following topical areas:
Internet Economics: auction theory and algorithmic game theory relevant to online advertising auctions.
•Cloud Computing: storage, databases, and optimization for computing in a massively distributed environment.
•Social Computing: models, algorithms and systems around social networks, social media, social search and collaborative environments.
•Data Mining and Machine Learning: learning algorithms, feature generation, and evaluation methods to produce effective online and offline models of behavioral signals.
•Systems: Hardware, operating system, runtime, and language support for fast, scalable, efficient data centers.
•Information Retrieval: search algorithms, information extraction, question answering, cross-lingual retrieval and multimedia retrieval
Eligibility Criteria
•Full-time Ph.D. students in topical areas represented by these fellowships who are currently involved in on-going research.
•Students must be in Computer Science, Computer Engineering, Electrical Engineering, System Architecture, or a related area.
•Students must be enrolled during the academic year that the Fellowship is awarded.
•Students must be nominated by a faculty member.
For more information (include the details of what's required to apply and how much cash it's worth), check out Facebook's Fellowship page. Application deadline is February 15th.
Tip to Barbara Kimbell.
posted January 8, 2010 06:26 PM in Simply Academic | permalink | Comments (0)
December 07, 2009
With boundless delight
While visiting Petter Holme in Korea last week, we naturally engaged in a little bit of that favorite past time of researchers: griping about cowardly editors, capricious referees, and how much publishing sometimes seems like a popularity contest. In the midst of this, Petter mentioned an old gem of a rejection letter, now widely quoted on the Internets, but new to me.
This apocryphal rejection was apparently quoted in the Chronicle of Higher Education several years ago, but I couldn't find it in the Chronicle's archives; it's also rumored to have been quoted in the Financial Times, but I couldn't find it in their archives, either. Here's a version of it, with some editorial commentary from another source, that Petter shared with me:
Responses from several journal editors seek to hearten authors by noting that an article's rejection may constitute neither a personal rebuke nor disparagement of the article's ideas. However, the following rejection letter from a Chinese economics journal inflicts the same damage as a blunt, two-sentence refusal: "We have read your manuscript with boundless delight. If we were to publish your paper, it would be impossible for us to publish any work of lower standard. And as it is unthinkable that in the next thousand years we shall see its equal, we are, to our regret, compelled to return your divine composition, and to beg you a thousand times to overlook our short sight and timidity."
A beautifully backhanded rejection, but it smells a little like an urban ("academic"?) myth. Petter pointed out that a Chinese journal would more probably have said "beg you ten thousand times to overlook" rather than just a "thousand times". This stems from the cultural usage of "ten thousand" being equivalent to a very large but unspecified number, while "a thousand" typically just means 1000. If the original rejection was in Chinese, this could simply be a translation error. Or, the Chinese journal might have been being especially mean spirited.
Update 7 December 2009: Dave Schwab points me to a video Cosma Shalizi sent me a few weeks ago, about peer review. Despite the obviously terrible German-to-English translation, it does a pretty good job of summarizing many people's feelings about the vagrancies of the peer review process.
Update 8 December 2009: For many of us with fingers (or whole selves) in the computer science world, it's reviewing season for several conferences. This year, I'm on the program committee for the WWW 2010 conference. Inside some of the recent PC-related emails, there was a link to a brief, tongue-in-cheek article about How NOT to review a paper, in which Graham Cormode of AT&T describes the tools of the "adversarial reviewer." Having recently experienced some of these very tactics (with a paper submitted to PNAS), it's a fun read. I particularly liked his future direction in which he (half) advocates turning reviewing into a blood sport. Too late!
posted December 7, 2009 08:59 AM in Simply Academic | permalink | Comments (2)
October 24, 2009
This is the life I've chosen
An oldie, but goodie: John Oliver reporting on how academia really works.
The Daily Show With Jon Stewart | Mon - Thurs 11p / 10c | |||
Human's Closest Relative | ||||
|
If that's not enough hilarity about chimps vs. orangs, or, if you were really intrigued by the arguments in favor of orangs, read this.
Tip to Jake Hofman.
posted October 24, 2009 09:45 AM in Simply Academic | permalink | Comments (0)
January 26, 2009
The right place for science
Dennis Overbye has a very nice little essay in the Science Times this week on the restoration of science to its rightful place in society, and on the common themes that make both science and democracy function. Here's a blurb:
Science is not a monument of received Truth but something that people do to look for truth.
That endeavor, which has transformed the world in the last few centuries, does indeed teach values. Those values, among others, are honesty, doubt, respect for evidence, openness, accountability and tolerance and indeed hunger for opposing points of view. These are the unabashedly pragmatic working principles that guide the buzzing, testing, poking, probing, argumentative, gossiping, gadgety, joking, dreaming and tendentious cloud of activity — the writer and biologist Lewis Thomas once likened it to an anthill — that is slowly and thoroughly penetrating every nook and cranny of the world.
Nobody appeared in a cloud of smoke and taught scientists these virtues. This behavior simply evolved because it worked.
This sounds pretty good, doesn't it? And I think it's basically true (although not necessarily in the way we might naively expect) that aspects of science are pervading almost every part of modern life and thought. One thing that I've found particularly bizarre in recent years is the media's promotion of words like "Why" and "How" to a pole position in their headlines. For instance, Time Magazine now routinely blasts "How such and such happens" across its front page, suggesting that within its pages, definitive answers for the mysteries of life will be revealed. To me, this apes the way scientists often talk, and capitalizes on society's susceptibility to that kind of language. If science weren't such a dominant force in our society, this kind of tactic would surely not sell magazines...
posted January 26, 2009 10:28 PM in Simply Academic | permalink | Comments (0)
October 23, 2007
SFI is hiring
From personal experience, I can attest to the fact that SFI is a great place to work, do science, learn stuff, explore new areas, and otherwise build your career. Start your LaTeX engines! (Deadline is Nov. 15, a scant 3 weeks away!)
Postdoctoral Fellowship Opportunities at the Santa Fe Institute
The Santa Fe Institute (SFI) is selectively seeking applications for Postdoctoral Fellows for appointments beginning Fall 2008.
Fellows are appointed for up to three years during which they pursue research questions of their own design and are encouraged to transcend disciplinary lines. SFI’s unique structure and resources enable Fellows to collaborate with members of the SFI faculty, other Fellows, and researchers from around the world.
As the leader in multidisciplinary research, SFI has no formal programs or departments and we accept applications from any field. Research topics span the full range of natural and social sciences and often make connections with the humanities. Most research at SFI is theoretical and/or computational in nature, although some research includes an empirical component in collaboration with other institutions.
The compensation package includes a competitive salary and excellent health and retirement benefits. As full participants in the SFI community, Fellows are encouraged to invite speakers, organize workshops and working groups and engage in research outside their field. Funds are available to support this full range of research activities. Applications are welcome from candidates in any country. Successful foreign applicants must acquire an acceptable visa (usually a J-1) as a condition of employment. Women and minorities are especially encouraged to apply.
For complete information and application instructions, please follow the link to http://www.santafe.edu/postdocapp08.
The online application process opens October 15, 2007. Application deadline is November 15, 2007.
posted October 23, 2007 06:22 PM in Simply Academic | permalink | Comments (0)
April 15, 2007
A rose is a rose
Warning: Because I'm still recovering from my catastrophic loss last Monday, blogging will be light or ridiculous for a little while longer. So, without further ado...
A few weeks ago, I inadvertently initiated a competition in the comment thread of Scott Aaronson's blog on how to identify physicists. It all started with Scott claiming that he was not a mathematician (as New Scientist claimed he was in an article about D-wave's press releases about quantum computers). As various peoples weighed in on Scott's mathematicianness, finally Dave Bacon proposed a sure fire way to settle the question:
Place yourself and a large potted plant in a huge room together. If you get tangled up in the plant, you are a mathematician. I draw this test from careful observation of the MSRI in Berkeley.
I then wondered aloud how to identify physicists, and I was returned a laundry list of characteristic behaviors:
- Hearing the word “engineering” causes a skin rash. [John Sidles]
- Writes “a”, says “b”, means “c”, but it should be “d” [Polya]
- Frequently begins sentences with “As a physicist…” (as in “As a physicist, I care about the real world, not the logical consequences of the assumption I just made”) [Scott Aaronson]
- When told he is actually a mathematician he thinks: “LOL” and all the mathematician go: “OMFG”. [Peter Sheldrick]
- They think that, since walking forwards gets them from their house to work, walking backwards in the opposite direction must have the same outcome. (Re: the replica method) [James]
- Is interested in creating just one job. [John Sidles]
- Considers chemists to be underqualified physicists, and biologists to be overqualified philatelists. [anonymous]
Amusingly, I know many people (physicists, mostly) who are walking, talking caricature of these. I also know some excellent people in physics departments who certainly are not, and I'm not sure what they do is "physics". I wonder if they think of themselves as physicists...
I know I promised to keep this ridiculous, but I can hardly help myself. So, if you'll permit me a lengthy navel-gazing digression, there's an interesting question here, which has to do with the labels communities of people choose to adopt, and how they view interlopers. For instance, I have no idea whether to call myself an applied mathematician (maybe not), a physicist (almost certainly not, although most of my publications are in physics journals), a computer scientist (still not quite right even though my doctorate is in CS), or what. Informatician sounds like a career in oratory, no one knows what an "applied computer scientist" is, and none of Complex systemsatist, "compleximagician," or statico-phyico-algorithmo-informa-complexicist have that nifty ring to them. (And, for that matter, neither does plecticist.)
With my recent phase change, when people ask what I do, I've taken to simply saying that I'm a "scientist." But, that just encourages them to ask the obvious follow up: What kind of scientist? In some sense, applied mathematician seems colloquially, kind of, maybe, almost like what I do. But, I'm not sure I could teach in a mathematics department, nor would other applied mathematicians call me one of their own. Obviously, these labels are all artificial, but they do matter for hiring, publishing, and general academic success. The complex systems community hasn't achieved a critical-enough mass to assert its own labels for the people who seem to do that kind of work, so, in the meantime, how should we name the practitioners in this field?
Update, 16 April 2007: One colleague suggests "mathematical scientist" as an appropriate moniker, which I tend to also like. Sadly, I'm not sure other scientists would agree that this is a useful label, nor do I expect to see many Departments of Mathematical Science being created in the near future (and similarly for "computational scientist") ... End Update
posted April 15, 2007 08:30 PM in Humor | permalink | Comments (8)
March 31, 2007
arXiv phase change
The arXiv has been discussing for some time the need to change the way it tags submissions. The principal motivation was that the number of monthly submissions in some subject classes (math and cond-mat, for instance) has been steadily rising over the past few years, and would have likely crossed the break-point of 1000 per month sometime later this year. 1000 is the magic number because the current arxiv tag is formatted as "subject-class/YYMMNNN".
The new tagging system, which goes into effect for all submissions tomorrow April 1st and later, moves to a "YYMM.NNNN" format and drops the subject classification prefix. I rather like this change, since, by decoupling the classification and the tag, it gives arXiv a lot more flexibility to adapt its internal subject classes to scientific trends. This will make it easier to place multi-disciplinary articles (like those I write, most of which end up on the physics arxiv), will (hopefully) make it less confusing for people to find articles, and will (potentially) let the arxiv expand into other scientific domains.
posted March 31, 2007 12:01 AM in Simply Academic | permalink | Comments (0)
March 29, 2007
Nemesis or Archenemy
Via Julianne of Cosmic Variance, the rules of the game for choosing your archnemesis. The rules are so great, I reproduce them here, in full.
1. Your archnemesis cannot be your junior. Someone who is in a weaker position than you is not worthy of being your archnemesis. If you designate someone junior as your archnemesis, you’re abusing your power.
2. You cannot have more than one archnemesis. Most of us have had run-ins with scientific groups who range continuous war against all outsiders. They take a scorched earth policy to anyone who is not a member of their club. However, while these people are worthy candidates for being your archnemesis, they are not allowed to have that many archnemeses themselves. If you find that many, many people are your archnemeses, then you’re either (1) paranoid; (2) an asshole; or (3) in a subfield that is so poisonous that you should switch topics. If (1) or (2) is the case, tone it down and try to be a bit more gracious.
3. Your archnemesis has to be comparable to you in scientific ability. It is tempting to despise the one or two people in your field who seem to nab all the job offers, grants, and prizes. However, sometimes they do so because they are simply more effective scientists (i.e. more publications, more timely ideas, etc) or lucky (i.e. wound up discovering something unexpected but cool). If you choose one of these people as an archnemesis based on greater success alone, it comes off as sour grapes. Now, if they nabbed all the job offers, grants, and prizes because they stole people’s data, terrorized their juniors, and misrepresented their work, then they are ripe and juicy for picking as your archnemesis. They will make an even more satisfying archnemesis if their sins are not widely known, because you have the future hope of watching their fall from grace (not that this actually happens in most cases, but the possibility is delicious). Likewise, other scientists may be irritating because their work is consistently confusing and misguided. However, they too are not candidates for becoming your archnemesis. You need to take a benevolent view of their struggles, which are greater than your own. [Ed: Upon recovering my composure after reading this last line, I decided it is, indeed, extremely good advice.]
4. Archnemesisness is not necessarily reciprocal. Because of the rules of not picking fights with your juniors, you are not necessarily your archnemesis’s archnemesis. A senior person who has attempted to cut down a grad student or postdoc is worthy of being an archnemesis, but the junior people in that relationship are not worthy of being the archnemesis of the senior person. There’s also the issue that archnemeses are simply more evil than you, so while they’ll work hard to undermine you, you are sufficiently noble and good that you would not actively work to destroy them (though you would smirk if it were to happen).
Now, what does one do with an archnemesis? Nothing. The key to using your archnemesis effectively is to never, ever act as if they’re your archnemesis (except maybe over beers with a few close friends when you need to let off steam). You do not let yourself sink to their level, and take on petty fights. You do not waste time obsessing about them. Instead, you treat them with the same respect that you would any other colleague (though of course never letting them into a position where they could hurt you, like dealing with a cobra). You only should let your archnemesis serve as motivation to keep pursuing excellence (because nothing annoys a good archnemesis like other people’s success) and as a model of how not to act towards others. You’re allowed to take private pleasure in their struggles or downfall, but you must not ever gloat.
While I’m sure the above sounds so thrilling that you want to rush out and get yourself an archnemesis, if one has not been thrust upon you, count your blessings. May your good fortune continue throughout your career.
In the comment thread, bswift points to a 2004 Esquire magazine piece by Chuck Klosterman on the difference between your (arch)nemesis and your archenemy. Again, quoting liberally.
Now, I know that you’re probably asking yourself, How do I know the difference between my nemesis and my archenemy? Here is the short answer: You kind of like your nemesis, despite the fact that you despise him. If your nemesis invited you out for cocktails, you would accept the offer. If he died, you would attend his funeral and—privately—you might shed a tear over his passing. But you would never have drinks with your archenemy, unless you were attempting to spike his gin with hemlock. If you were to perish, your archenemy would dance on your grave, and then he’d burn down your house and molest your children. You hate your archenemy so much that you try to keep your hatred secret, because you don’t want your archenemy to have the satisfaction of being hated.
Naturally I wonder, Do I have an archnemesis, or an archenemy? Over the years, I've certainly had a few adversarial relationships, and many lively sparring matches, with people at least as junior as me, but they've never been driven by the same kind of deep-seated resentment, and general bad behavior, that these two categories seem to require. So, I count myself lucky that in the fictional story of my life, I've had only "benign" professional relationships - that is, the kind disqualified from nemesis status. However, on the (quantum mechanical) chance that my fictional life takes a dramatic turn, and a figure emerges to play the Mr. Burns to my Homer Simpson, the Newman to my Seinfeld, the Dr. Evil to my Austin Powers, I'll keep these rules (and that small dose of hemlock) handy.
Update, March 30, 2007: Over in the comment section, I posed the question of whether Feynman was Gell-Mann's archnemesis, as I suspected. Having recently read biographies of both men (here and here), it was hard to ignore the subtle (and not-so-subtle) digs that each man made at the other through these stories. A fellow commenter Elliot, who was at Caltech when Gell-Mann received his Nobel confirmed that Feynman was indeed Gell-Mann's archnemesis, not for scientific reasons, but for social ones. Looking back over the rules of the game, Feynman does indeed satisfy all the criteria. Cute.
posted March 29, 2007 12:04 AM in Simply Academic | permalink | Comments (0)
February 17, 2007
What makes a good (peer) reviewer?
The peer review process is widely criticized for its failings, and many of them are legitimate complaints. But, to paraphrase Churchill, No one pretends that peer review is perfect or all-wise. In fact, peer review is the worst of all systems, except for all the others. Still, peer review is itself only occasionally studied. So, that makes the work of two medical researchers all the more interesting. Callaham and Tercier studied about 3000 reviews of about 1500 manuscripts by about 300 reviewers over a four-year period, and the corresponding quality scores given these reviews by editors.
Our study confirms that there are no easily identifiable types of formal training or experience that predict reviewer performance. Skill in scientific peer review may be as ill defined and hard to impart as is “common sense.” Without a better understanding of those skills, it seems unlikely journals and editors will be successful in systematically improving their selection of reviewers. This inability to predict performance makes it imperative that all but the smallest journals implement routine review ratings systems to routinely monitor the quality of their reviews (and thus the quality of the science they publish).
The other choice results of their study include a consistent negative correlation between the quality of the review and the number of years of experience. That is, younger reviewers write better reviews. To anyone in academia, this should be a truism for obvious reasons. Ironically, service on an Institutional Review Board (IRB; permission from such a board is required to conduct experiments with human subjects) consistently correlated with lower-quality reviews. The caveat here, of course, is that both these and the other factors were only slightly significant.
I've been reviewing for a variety of journals and conferences (across Computer Science, Physics, Biology and Political Science) for a number of years now, and I still find myself trying to write thoughtful, and sometimes lengthy, reviews. I think this is because I honestly believe in the system of peer review, and always appreciate thoughtful reviews myself. Over the years, I've changed some things about how I review papers. I often start earlier now, write a first draft of the review, and then put it down for several days. This lets my thoughts settle on the important points of the paper, rather than on the details that jump out initially. If the paper is good, I try to make small constructive suggestions. If the paper isn't so good, I try to point out the positive aspects, and couch my criticism on firm scientific grounds. In both, I try to see the large context that the results fit into. For some manuscripts, these things are harder than others, particularly if the work seems to have been done hastily, the methodology suspect or poorly described, the conclusions overly broad, etc. My hope is that, once I have a more time-consuming position, I'll have developed some tricks and habits that let me continue to be thoughtful in my reviews, but able to spend less time doing them.
Callaham and Tercier, "The Relationship of Previous Training and Experience of Journal Peer Reviewers to Subsequent Review Quality." PLoS Medicine 4(1): e40 (2007).
Tip to Ars Technica, which has its own take on the study.
posted February 17, 2007 04:47 PM in Simply Academic | permalink | Comments (0)
January 25, 2007
DIMACS - Complex networks and their applications (Day 3)
The third day of the workshop focused on applications to biochemical networks (no food webs), with a lot of that focus being on the difficulties of taking fuzzy biological data (e.g., gene expression data) and converting it into an accurate and meaningful form for further analysis or for hypothesis testing. Only a few of the talks were theoretical, but this perhaps reflects the current distribution of focus in biology today. After the workshop was done, I wondered just how much information crossed between the various disciplines represented at the workshop - certainly, I came away from it with a few new ideas, and a few new insights from the good talks I attended. And I think that's the sign of a successful workshop.
Complex Networks in Biology
Chris Wiggins (Columbia) delivered a great survey of interesting connections between machine learning and biochemical networks. It's probably fair to say that biologists are interested in constructing an understanding of cellular-level systems that compares favorably to an electrical engineer's understanding of circuits (Pointer: Can a Biologist Fix a Radio?). But, this is hard because living stuff is messy, inconsistent in funny ways, and has a tendency to change while you're studying it. So, it's harder to get a clean view of what's going on under the hood than it was with particle physics. This, of course, is where machine learning is going to save us - ML offers powerful and principled ways to sift through (torture) all this data.
The most interesting part of his talk, I think, was his presentation of NetBoost, a mechanism discriminator that can tell you which (among a specific suite of existing candidates) is the most likely to have generated your observed network data [1]. For instance, was it preferential attachment (PA) or duplication-mutation-complementation (DMC) that produced a given protein-interaction network (conclusion: the latter is better supported). The method basically works by constructing a decision tree that looks at the subgraph decomposition of a network and scores it's belief that each of the various mechanisms produced it [2]. With the ongoing proliferation of network mechanisms (theorists really don't have enough to do these days), this kind of approach serves as an excellent way to test a new mechanism against the data it's supposed to be emulating.
One point Chris made that resonated strongly with me - and which Cris and Mark made yesterday - is the problem with what you might call "soft validation" [3]. Typically, a study will cluster or do some other kind of analysis with the data, and then tell a biological story about why these results make sense. On the other hand, forcing the clustering to make testable predictions would be a stronger kind of validation.
Network Inference and Analysis for Systems Biology
Just before lunch, Joel Bader (Johns Hopkins) gave a brief talk about his work on building a good view of the protein-protein interaction network (PPIN). The main problems with this widely studied data are the high error rate, both for false positives (interactions that we think exist, but don't) and false negatives (interactions that we think don't exist, but do). To drive home just how bad the data is, he pointed out that two independent studies of the human PPIN showed just 1% overlap in the sets of "observed" interactions.
He's done a tremendous amount of work on trying to improve the accuracy of our understanding of PPINs, but here he described a recent approach that fits degree-based generative models [4] to the data using our old friend expectation-maximization (EM) [5]. His results suggest that we're seeing about 30-40% of the real edges, but that our false positive rate is about 10-15%. This is a depressing signal-to-noise ratio (roughly 1%), because the number of real interactions is O(n), while our false positive rate is O(n^2). Clearly, the biological methods used to infer the interactions need to be improved before we have a clear idea of what this network looks like, but it also suggests that a lot of the previous results on this network are almost surely wrong. Another question is whether it's possible to incorporate these kinds of uncertainties into our analyses of the network structure.
Activating Interaction Networks and the Dynamics of Biological Networks
Meredith Betterton (UC-Boulder) presented some interesting work on signaling and regulatory networks. One of the more surprising tidbits she used in her motivation is the following. In yeast, the mRNA transcription undergoes a consistent 40-minute genome-wide oscillation, but when exposed to an antidepressant (in this case, phenelzine), the period doubles [6]. (The fact that gene expression oscillates like this poses another serious problem for the results of gene expression analysis that doesn't account for such oscillations.)
The point Meredith wanted to drive home, though, was we shouldn't just think of biochemical networks as static objects - they also represent the form that the cellular dynamics must follow. Using a simple dynamical model of activation and inhibition, she showed that the structure (who points to who, and whether an edge inhibits or activates its target) of a real-world circadian rhythm network and a real-world membrane-based signal cascade basically behave exactly as you would expect - one oscillates and the other doesn't. But, then she showed that it only takes a relatively small number of flips (activation to inhibition, or vice versa) to dramatically change the steady-state behavior of these cellular circuits. In a sense, this suggests that these circuits are highly adaptable, given a little pressure.
There are several interesting questions that came to mind while she was presenting. For instance, if we believe there are modules within the signaling pathways that accomplish a specific function, how can we identify them? Do sparsely connected dense subgraphs (assortative community structure) map onto these functional modules? What are the good models for understanding these dynamics, systems of differential equations, discrete time and matrix multiplication, or something more akin to a cellular version of Ohm's Law? [7]
-----
[1] M. Middendorf, E. Ziv and C. Wiggins, "Inferring Network Mechanisms: The Drosophila melanogaster Protein Interaction Network." PNAS USA 102 (9), 3192 (2005).
[2] Technically, it's using these subgraphs as generic features and then crunching the feature vectors from examples of each mechanism through a generalized decision tree in order to learn how to discriminate among them. Boosting is used within this process in order to reduce the error rates. The advantage of this approach to model selection and validation, as Chris pointed out, is that it doesn't assume a priori which features (e.g., degree distribution, clustering coefficient, distance distribution, whatever) are interesting, but rather chooses the ones that can actually discriminate between things we believe are different.
[3] Chris called it "biological validation," but the same thing happens in sociology and Internet modeling, too.
[4] I admit that I'm a little skeptical of degree-based models of these networks, since they seem to assume that we're getting the degree distribution roughly right. That assumption is only reasonable if our sampling of the interactions attached to a particular vertex is unbiased, which I'm not sure about.
[5] After some digging, I couldn't find the reference for this work. I did find this one, however, which illustrates a different technique for a related problem. I. Iossifov et al., "Probabilistic inference of molecular networks from noisy data sources." 20 (8), 1205 (2004).
[6] C. M. Li and R. R. Klevecz, "A rapid genome-scale response of the transcriptional oscillator to perturbation reveals a period-doubling path to phenotypic change." PNAS USA 103 (44), 16254 (2006).
[7] Maribeth Oscamou pointed out to me during the talk that any attempt to construct such rules have to account for processes like the biochemical degradation of the signals. That is, unlike electric circuits, there's no strict conservation of the "charge" carrier.
posted January 25, 2007 01:20 PM in Scientifically Speaking | permalink | Comments (0)
January 24, 2007
DIMACS - Complex networks and their applications (Day 2)
There were several interesting talks today, or rather, I should say that there were several talks today that made me think about things beyond just what the presenters said. Here's a brief recap of the ones that made me think the most, and some commentary about what I thought about. There were other good talks today, too. For instance, I particularly enjoyed Frank McSherry's talk on doing PageRank on his laptop. There was also one talk on power laws and scale-free graphs that stimulated a lot of audience, ah, interaction - it seems that there's a lot of confusion both over what a scale-free graph is (admittedly the term has no consistent definition in the literature, although there have been some recent attempts to clarify it in a principled manner), and how to best show that some data exhibit power-law behavior. Tomorrow's talks will be more about networks in various biological contexts.
Complex Structures in Complex Networks
Mark Newman's (U. Michigan) plenary talk mainly focused on the importance of having good techniques to extract information from networks, and being able to do so without making a lot of assumptions about what the technique is supposed to look for. That is, rather than assume that some particular kind of structure exists and then look for it in our data, why not let the data tell you what kind of interesting structure it has to offer? [1] The tricky thing about this approach to network analysis, though, is working out a method that is flexible enough to find many different kinds of structure, and to present only that which is unusually strong. (Point to ponder: what should we mean by "unusually strong"?) This point was a common theme in a couple of the talks today. The first example that Mark gave of a technique that has this nice property was a beautiful application of spectral graph theory to the task of find a partition of the vertices that give an extremal value of modularity. If we ask for the maximum modularity, this heuristic method [2], using the positive eigenvalues of the resulting solution, gives us a partition with very high modularity. But, using the negative eigenvalues gives a partition that minimizes the modularity. I think we normally think of modules meaning assortative structures, i.e., sparsely connected dense subgraphs. But, some networks exhibit modules that are approximately bipartite, i.e., they are disassortative, being densely connected sparse subgraphs. Mark's method naturally allows you to look for either. The second method he presented was a powerful probabilistic model of node clustering that can be appropriately parameterized (fitted to data) via expectation-maximization (EM). This method can be used to accomplish much the same results as the previous spectral method, except that it can look for both assortative and disassortative structure simultaneously in the same network.
Hierarchical Structure and the Prediction of Missing Links
In an afternoon talk, Cris Moore (U. New Mexico) presented a new and powerful model of network structure, the hierarchical random graph (HRG) [5]. (Disclaimer: this is joint work with myself and Mark Newman.) A lot of people in the complex networks literature have talked about hierarchy, and, presumably, when they do so, they mean something roughly along the lines of the HRG that Cris presented. That is, they mean that nodes with a common ancestor low in the hierarchical structure are more likely to be connected to each other, and that different cuts across it should produce partitions that look like communities. The HRG model Cris presented makes these notions explicit, but also naturally captures the kind of assortative hierarchical structure and the disassortative structure that Mark's methods find. (Test to do: use HRG to generate mixture of assortative and disassortative structure, then use Mark's second method to find it.) There are several other attractive qualities of the HRG, too. For instance, using a Monte Carlo Markov chain, you can find the hierarchical decomposition of a single real-world network, and then use the HRG to generate a whole ensemble of networks that are statistically similar to the original graph [6]. And, because the MCMC samples the entire posterior distribution of models-given-the-data, you can look not only at models that give the best fit to the data, but you can look at the large number of models that give an almost-best fit. Averaging properties over this ensemble can give you more robust estimates of unusual topological patterns, and Cris showed how it can also be used to predict missing edges. That is, suppose I hide some edges and then ask the model to predict which ones I hid. If it can do well at this task, then we've shown that the model is capturing real correlations in the topology of the real graph - it has the kind of explanatory power that comes from making correct predictions. These kinds of predictions could be extremely useful for laboratory or field scientists who manually collect network data (e.g., protein interaction networks or food webs) [7]. Okay, enough about my own work!
The Optimization Origins of Preferential Attachment
Although I've seen Raissa D'Souza (UC Davis) talk about competition-induced preferential attachment [8] before, it's such an elegant generalization of PA that I enjoyed it a second time today. Raissa began by pointing out that most power laws in the real-world can't extend to infinity - in most systems, there are finite limits to the size that things can be (the energy released in an earthquake or the number of edges a vertex can have), and these finite effects will typically manifest themselves as exponential cutoffs in the far upper tail of the distribution, which takes the probability of these super-large events to zero. She used this discussion as a springboard to introduce a relatively simple model of resource constraints and competition among vertices in a growing network that produces a power-law degree distribution with such an exponential cutoff. The thing I like most about this model is that it provides a way for (tempered) PA to emerge from microscopic and inherently local interactions (normally, to get pure PA to work, you need global information about the system). The next step, of course, is to find some way to measure evidence for this mechanism in real-world networks [9]. I also wonder how brittle the power-law result is, i.e., if you tweak the dynamics a little, does the power-law behavior disappear?
Web Search and Online Communities
Andrew Tomkins (of Yahoo! Reserch) is a data guy, and his plenary talk drove home the point that Web 2.0 applications (i.e., things that revolve around user-generated content) are creating a huge amount of data, and offering unparalleled challenges for combining, analyzing, and visualizing this data in meaningful ways. He used Flickr (a recent Y! acquisition) as a compelling example by showing an interactive (with fast-rewind and fast-forward features) visual stream of the trends in user-generated tags for user-posted images, annotated with notable examples of those images. He talked a little about the trickiness of the algorithms necessary to make such an application, but what struck me most was his plea for help and ideas in how to combine information drawn from social networks with user behavior with blog content, etc. to make more meaningful and more useful applications - there's all this data, and they only have a few ideas about how to combine it. The more I learn about Y! Research, the more impressed I am with both the quality of their scientists (they recently hired Duncan Watts), and the quality of their data. Web 2.0 stuff like this gives me the late-1990s shivers all over again. (Tomkins mentioned that in Korea, unlike in the US, PageRank-based search has been overtaken by an engine called Naver, which is driven by users building good sets of responses to common search queries.)
-----
[1] To be more concrete, and perhaps in lieu of having a better way of approaching the problem, much of the past work on network analysis has taken the following approach. First, think of some structure that you think might be interesting (e.g., the density of triangles or the division into sparsely connected dense subgraphs), design a measure that captures that structure, and then measure it in your data (it turns out to be non-trivial to do this in an algorithm independent way). Of course, the big problem with this approach is that you'll never know whether there is other structure that's just as important as, or maybe more important than, the kind you looked for, and that you just weren't clever enough to think to look for it.
[2] Heuristic because Mark's method is a polynomial time algorithm, while the problem of modularity maximization was recently (finally...) shown to be NP-complete. The proof is simple, and, in retrospect, obvious - just as most such proofs inevitably end up being. See U. Brandes et al. "Maximizing Modularity is hard." Preprint (2006).
[3] M. E. J. Newman, "Finding community structure in networks using the eigenvectors of matrices." PRE 74, 036104 (2006).
[4] M. E. J. Newman and E. A. Leicht, "Mixture models and exploratory data analysis in networks." Submitted to PNAS USA (2006).
[5] A. Clauset, C. Moore and M. E. J. Newman, "Structural Inference of Hierarchies in Networks." In Proc. of the 23rd ICML, Workshop on "Statistical Network Analysis", Springer LNCS (Pittsburgh, June 2006).
[6] This capability seems genuinely novel. Given that there are an astronomical number of ways to rearrange the edges on a graph, it's kind of amazing that the hierarchical decomposition gives you a way to do such a rearrangement, but one which preserves the statistical regularities in the original graph. We've demonstrated this for the degree distribution, the clustering coefficient, and the distribution of pair-wise distances. Because of the details of the model, it sometimes gets the clustering coefficient a little wrong, but I wonder just how powerful / how general this capability is.
[7] More generally though, I think the idea of testing a network model by asking how well it can predict things about real-world problems is an important step forward for the field; previously, "validation" consisted of showing only a qualitative (or worse, a subjective) agreement between some statistical measure of the model's behavior (e.g., degree distribution is right-skewed) and the same statistical measure on a real-world network. By being more quantitative - by being more stringent - we can say stronger things about the correctness of our mechanisms and models.
[8] R. M. D'Souza, C. Borgs, J. T. Chayes, N. Berger, and R. Kleinberg, "Emergence of Tempered Preferential Attachment From Optimization", To appear in PNAS USA, (2007).
[9] I think the best candidate here would be the BGP graph, since there is clearly competition there, although I suspect that the BGP graph structure is a lot more rich than the simple power-law-centric analysis has suggested. This is primarily due to the fact that almost all previous analyses have ignored the fact that the BGP graph exists as an expression of the interaction of business interests with the affordances of the Border Gateway Protocol itself. So, its topological structure is meaningless without accounting for the way it's used, and this means accounting for complexities of the customer-provider and peer-to-peer relationships on the edges (to say nothing of the sampling issues involved in getting an accurate BGP map).
posted January 24, 2007 02:18 AM in Scientifically Speaking | permalink | Comments (1)
January 23, 2007
DIMACS - Complex networks and their applications (Day 1)
Today and tomorrow, I'm at the DIMACS workshop on complex networks and their applications, held at Georgia Tech's College of Computing. Over the course of the workshop, I'll be blogging about the talks I see and whatever ideas they stimulate (sadly, I missed most of the first day because of travel).
The most interesting talk I saw Monday afternoon was by Ravi Kumar (Yahoo! Research), who took location data of users on LiveJournal, and asked Do we see the same kind of routable structure - i.e., an inverses-square law relationship in the distance between people and the likelihood that they have a LJ connection - that Kleinberg showed was optimal for distributed / local search? Surprisingly, they were able to show that in the US, once you correct for the fact that there can be many people at a single "location" in geographic space (approximated to the city level), you do indeed observe exactly the kind of power-law that Kleinberg predicted [1]. Truly, this was a kind of stunning confirmation of Kleinberg's theory. So now, the logical question would be, What mechanism might produce this kind of structure in geographic space? Although you could probably get away with assuming a priori the population distribution, what linking dynamics would construct the observed topological pattern? My first project in graduate school asked exactly this question for the pure Kleinberg model, and I wonder if it could be adapted to the geographic version that Kumar et al. consider.
[1] D. Liben-Nowell, et al. "Geographic Routing in Social Networks." PNAS USA 102, 33 11623-1162 (2005).
posted January 23, 2007 06:24 AM in Scientifically Speaking | permalink | Comments (0)
December 19, 2006
Phase change
This past weekend I graduated with distinction with my doctorate from the University of New Mexico's Department of Computer Science. My advisor Cristopher Moore hooded me at the main Commencement ceremony on Friday, and on Saturday, the School of Engineering had its own smaller (and nicer) Convocation ceremony for its graduates. I was invited to be the graduate speaker at this event, and I made a few brief remarks that you can read here.
It's been an intense and highly educational four and a half years, but it's nice to finally be done.
posted December 19, 2006 12:36 PM in Self Referential | permalink | Comments (1)
September 08, 2006
Academic publishing, tomorrow
Imagine a world where academic publishing is handled purely by academics, rather than ruthless, greedy corporate entities. [1] Imagine a world where hiring decisions were made on the techincal merit of your work, rather than the coterie of journals associated with your c.v. Imagine a world where papers are living documents, actively discussed and modified (wikified?) by the relevant community of interested intellectuals. This, and a bit more, is the future, according to Adam Rogers, a senior associate editor at "Wired" magazine. (tip to The Geomblog)
The gist of Rogers' argument is that the Web will change academic publishing into this utopian paradise of open information. I seriously doubt things will be like he predicts, but he does raise some excellent points about how the Web is facilitating new ways of communicating technical results. For instance, he mentions a couple of on-going experiments in this area:
In other quarters, traditional peer review has already been abandoned. Physicists and mathematicians today mainly communicate via a Web site called arXiv. (The X is supposed to be the Greek letter chi; it's pronounced "archive." If you were a physicist, you'd find that hilarious.) Since 1991, arXiv has been allowing researchers to post prepublication papers for their colleagues to read. The online journal Biology Direct publishes any article for which the author can find three members of its editorial board to write reviews. (The journal also posts the reviews – author names attached.) And when PLoS ONE launches later this year, the papers on its site will have been evaluated only for technical merit – do the work right and acceptance is guaranteed.
It's a bit hasty to claim that peer review has been "abandoned", but the arxiv has certainly almost completely supplanted some journals in their role of disseminating new research [2]. This is probably most true for physicists, since they're the ones who started the arxiv; other fields, like biology, don't have a pre-print archive (that I know of), but they seem to be moving toward open access journals for the same purpose. In computer science, we already have something like this, since the primary venue for publication is in conferences (which are peer reviewed, unlike conference in just about every other discipline), and whose papers are typically picked up by CiteSeer.
It seems that a lot of people are thinking or talking about open access this week. The Chronicle of Higher Education has a piece on the momentum for greater open access journals. It's main message is the new letter, signed by 53 presidents of liberal arts colleges (including my own Haverford College) in support of the bill currently in Congress (although unlikely to pass this year) that would mandate that all federally funded research be eventually made publicly available. The comments from the publishing industry are unsurprisingly self-interested and uninspiring, but they also betray a great deal of arrogance and greed. I wholeheartedly support more open access to articles - publicly funded research should be free to the public, just like public roads are free for everyone to use.
But, the bigger question here is, Could any these various alternatives to the pay-for-access model really replace journals? I'm less sure of the future here, as journals also serve a couple of other roles that things like the arxiv were never intended to fill. That is, journals run the peer review process, which, at its best, prevents erroneous research from getting a stamp of "community approval" and thereby distracting researchers for a while as they a) figure out that it's mistaken, and b) write new papers to correct it. This is why, I think, there is a lot of crap on the arxiv. A lot of authors self-police themselves quite well, and end up submitting nearly error-free and highly competent work to journals, but the error-checking process is crucial, I think. Sure, peer review does miss a lot of errors (and frauds), but, to paraphrase Mason Porter paraphrasing Churchill on democracy, peer review is the worst form of quality control for research, except for all the others. The real point here is that until something comes along that can replace journals as being the "community approved" body of work, I doubt they'll disappear. I do hope, though, that they'll morph into more benign organizations. PNAS and PLoS are excellent role models for the future, I think. And, they also happen to publish really great research.
Another point Rogers makes about the changes the Web is encouraging is a social one.
[...] Today’s undergrads have ... never functioned without IM and Wikipedia and arXiv, and they’re going to demand different kinds of review for different kinds of papers.
It's certainly true that I conduct my research very differently because I have access to Wikipedia, arxiv, email, etc. In fact, I would say that the real change these technologies will have on the world of research will be to decentralize it a little. It's now much easier to be a productive, contributing member of a research community without being down the hall from your colleagues and collaborators than it was 20 years ago. These electronic modes of communication just make it easier for information to flow freely, and I think that ultimately has a very positive effect on research itself. Taking that role away from the journals suggests that they will become more about getting that stamp of approval, than anything else. With its increased relative importance, who knows, perhaps journals will do a better job at running the peer review process (they could certainly use the Web, etc. to do a better job at picking reviewers...).
(For some more thoughts on this, see a recent discussion of mine with Mason Porter.)
Update Sept. 9: Suresh points to a recent post of his own about the arxiv and the issue of time-stamping.
[1] Actually, computer science conferences, impressively, are a reasonable approximation to this, although they have their own fair share of issues.
[2] A side effect of the arXiv is that it presents tricky issues regarding citation, timing and proper attribution. For instance, if a research article becomes a "living" documents, proper citation becomes rather problematic. For instance, which version of an article do you cite? (Surely not all of them!) And, if you revise your article after someone posts a derivative work, are you obligated to cite it in your revision?
posted September 8, 2006 05:23 PM in Simply Academic | permalink | Comments (3)
August 12, 2006
Your academic-journal dollars at work
Having now returned from a relaxing and rejuvenating trip to a remote (read: no Internet) beach with my family, I am trying to catch up on where the world has moved since I last checked. Comfortably, it's still in one piece, although I'm not thrilled about the latest draconian attempts to scare people into feeling safe about flying in airplanes. Amazingly, only half of the 300 emails I received were spam, and what remained were relatively quickly dispatched. In catching up on science news, I find a new movement afoot to stop Elsevier - the ruthless, and notoriously over-priced, academic publishing house - from organizing arms fairs via one of its subsidiaries. Having recently watched the excellent documentary Why We Fight, on the modern military-industrial complex, this makes me a little concerned.
I've only refereed once for any Elsevier journal, and I now plan to never referee for any of them again. This idea is, apparently, not uncommon among other scientists, e.g., here, here and here. Charging exorbitant prices to under-funded academics who produce and vet the very same content being sold is one thing - exploitative, yes; deadly, no - but arms fairs are a whole different kind of serious. Idiolect is running a petition against this behavior.
Update, Aug. 24: Digging around on YouTube, I found this interview with Eugene Jarecki, the director of Why We Fight.
posted August 12, 2006 09:19 PM in Simply Academic | permalink | Comments (0)
July 10, 2006
That career thing
I'm sure this piece of advice to young scientists by John Baez (of quantum gravity fame) is old news now (3 years on). But, seeing as it was written before I was paying attention to this kind of stuff myself, and it seems like quite good advice, here is it, in a nutshell:
1. Read voraciously, ask questions, don't be scared of "experts", and figure out what are the good problems to work on in your field.
2. Go the most prestigious school, and work with the best possible advisor.
3. Publish often and publish stuff people will want to read (and cite).
4. Go to conferences and give good, memorable talks.
Looking back over my success, so far, I think I've done a pretty good job on most of these things. His advice about going to a prestigious place seems to be more about getting a good advisor - I suppose that in physics, being a very old field, the best advisors can only be found at the most prestigious places. But, I'm not entirely convinced that this is true for the interdisciplinary mashup, which includes complex networks and the other things I like to study, yet...
posted July 10, 2006 12:50 AM in Simply Academic | permalink | Comments (3)
April 16, 2006
The view from the top
Richard Hamming, of coding theory fame, gave a talk at Bell Labs in 1986 as a retrospective on his career and his insights into how to do great research. In it, he tells many amusing anecdotes of his time at Bell Labs, including how he and Shannon were office mates at the same time he was working on information theory, and why so many of the smart people he knew produced little great research by the end of their careers. A fascinating read.
Hamming on the subject of a researcher's drive:
You observe that most great scientists have tremendous drive. I worked for ten years with John Tukey at Bell Labs. He had tremendous drive. One day about three or four years after I joined, I discovered that John Tukey was slightly younger than I was. John was a genius and I clearly was not. Well I went storming into Bode's office and said, ``How can anybody my age know as much as John Tukey does?'' He leaned back in his chair, put his hands behind his head, grinned slightly, and said, ``You would be surprised Hamming, how much you would know if you worked as hard as he did that many years.'' I simply slunk out of the office!
On the topic of knowing the limitations of your theories:
Great scientists tolerate ambiguity very well. They believe the theory enough to go ahead; they doubt it enough to notice the errors and faults so they can step forward and create the new replacement theory. If you believe too much you'll never notice the flaws; if you doubt too much you won't get started. It requires a lovely balance. But most great scientists are well aware of why their theories are true and they are also well aware of some slight misfits which don't quite fit and they don't forget it.
The rest of his talk is more of the same, but with longer stories and amusing anecdotes.
posted April 16, 2006 10:37 PM in Simply Academic | permalink | Comments (0)
March 07, 2006
Running a conference (redux)
Once again, for the past eight months or so, I've been heavily involved in running a small conference. The second annual Computer Science UNM Student Conference (CSUSC) happened this past Friday and was, in every sense of the word, a resounding success. Originally, this little shindig was conceived as a way for students to show off their research to each other, to the faculty, and to folks at Sandia National Labs. As such, this year's forum was just as strong as last year's inaugural session, having ten well-done research talks and more than a dozen poster presentations. Our keynote address was delivered by the friendly and soft-spoken David Brooks (no, not that one) from Harvard University, on power efficiency in computing. (Naturally, power density has been an important constraint on computing for a long time.)
Having organized this conference twice now, I have a very healthy respect for how much time is involved in making such an event a success. Although most of one's time is spent making sure all the gears are turning at the proper speeds (which includes, metaphorically, keeping the wheels greased and free of obstructions) so that each part completes in time to hand-off to the next, I'm also happy with how much of a learning experience its seems to have been for everyone involved (including me). This year's success was largely due to the excellent and tireless work of the Executive Committee, while, I'm confident saying that, all of the little hiccoughs we encountered were oversights on my part. Perhaps next year, those things will be done better by my successor.
But, the future success of the CSUSC is far from guaranteed: the probability of a fatal dip in the inertia of student interest in organizing it is non-trivial. This is a risk, I believe, that every small venue faces, since there are only ever a handful of students interested in taking time away from their usual menu of research and course work to try their hand at professional service. I wonder, What fraction of researchers are ever involved in organizing a conference? Reviewing papers is a standard professional duty, but the level of commitment required to run a conference is significantly larger - it takes a special degree of willingness (masochism?) and is yet another of the many parts of academic life that you have to learn in the trenches. For the CSUSC, I simply hope that the goodness that we've created so far continues on for a few more years, and am personally just glad we had such a good run over the past two.
With this out of the way, my conference calendar isn't quite empty, and is already rapidly refilling. Concurrent to my duties to the CSUSC, I've also been serving on the Program Committee for the 5th International Workshop on Experimental Algorithms (WEA), a medium-sized conference on the design, analysis and implementation of algorithms. An interesting experience, in itself, in part for broadening my perspective on the kind of research being done in algorithms. In May, always my busiest month for conferences, I'll be attending two events on network science. The first is CAIDA's Workshop on Internet Topology (WIT) in San Diego, while the second is the NetSci 2006 in Bloomington, Indiana.
posted March 7, 2006 04:37 AM in Simply Academic | permalink | Comments (0)
February 21, 2006
Pirates off the Coast of Paradise
At the beginning of graduate school, few people have a clear idea of what area of research they ultimately want to get into. Many come in with vague or ill-informed notions of their likes and dislikes, most of which are due to the idiosyncrasies of their undergraduate major's curriculum, and perhaps scraps of advice from busy professors. For Computer Science, it seems that most undergraduate curricula emphasize the physical computer, i.e., the programming, the operating system and basic algorithm analysis, over the science, let alone the underlying theory that makes computing itself understandable. For instance, as a teaching assistant for an algorithms course during my first semester in grad school, I was disabused of any preconceptions when many students had trouble designing, carrying-out, and writing-up a simple numerical experiment to measure the running time of an algorithm as a function of its input size, and I distinctly remember seeing several minds explode (and, not in the Eureka! sense) during a sketch of Cantor's diagonalization argument. When you consider these anecdotes along with the flat or declining numbers of students enrolling in computer science, we have a grim picture of both the value that society attributes to Computer Science and the future of the discipline.
The naive inference here would be that students are (rightly) shying away from a field that serves little purpose to society, or to them, beyond providing programming talent for other fields (e.g., the various biological or medical sciences, or IT departments, which have a bottomless appetite for people who can manage information with a computer). And, with programming jobs being outsourced to India and China, one might wonder if the future holds anything but an increasing Dilbert-ization of Computer Science.
This brings us to a recent talk delivered by Prof. Bernard Chazelle (CS, Princeton) at the AAAS Annual Meeting about the relevance of the Theory of Computer Science (TCS for short). Chazelle's talk was covered briefly by PhysOrg, although his separate and longer essay really does a better job of making the point,
Moore's Law has fueled computer science's sizzle and sparkle, but it may have obscured its uncanny resemblance to pre-Einstein physics: healthy and plump and ripe for a revolution. Computing promises to be the most disruptive scientific paradigm since quantum mechanics. Unfortunately, it is the proverbial riddle wrapped in a mystery inside an enigma. The stakes are high, for our inability to “get” what computing is all about may well play iceberg to the Titanic of modern science.
He means that behind the glitz and glam of iPods, Internet porn, and unmanned autonomous vehicles armed with GPS-guided missles, TCS has been drawing fundamental connections, through the paradigm of abstract computation, between previously disparate areas throughout science. Suresh Venkatasubramanian (see also Jeff Erickson and Lance Fortnow) phrases it in the form of something like a Buddhist koan,
Theoretical computer science would exist even if there were no computers.
Scott Aaronson, in his inimitable style, puts it more directly and draws an important connection with physics,
The first lesson is that computational complexity theory is really, really, really not about computers. Computers play the same role in complexity that clocks, trains, and elevators play in relativity. They're a great way to illustrate the point, they were probably essential for discovering the point, but they're not the point. The best definition of complexity theory I can think of is that it's quantitative theology: the mathematical study of hypothetical superintelligent beings such as gods.
Actually, that last bit may be overstating things a little, but the idea is fair. Just as theoretical physics describes the physical limits of reality, theoretical computer science describes both the limits of what can be computed and how. But, what is physically possible is tightly related to what is computationally possible; physics is a certain kind of computation. For instance, a guiding principle of physics is that of energy minimization, which is a specific kind of search problem, and search problems are the hallmark of CS.
The Theory of Computer Science is, quite to the contrary of the impression with which I was left after my several TCS courses in graduate school, much more than proving that certain problems are "hard" (NP-complete) or "easy" (in P), or that we can sometimes get "close" to the best much more easily than we can find the best itself (approximation algorithms), or especially that working in TCS requires learning a host of seemingly unrelated tricks, hacks and gimmicks. Were it only these, TCS would be interesting in the same way that Sudoku puzzles are interesting - mildly diverting for some time, but eventually you get tired of doing the same thing over and over.
Fortunately, TCS is much more than these things. It is the thin filament that connects the mathematics of every natural science, touching at once game theory, information theory, learning theory, search and optimization, number theory, and many more. Results in TCS, and in complexity theory specifically, have deep and profound implications for what the future will look like. (E.g., do we live in a world where no secret can actually be kept hidden from a nosey third party?) A few TCS-related topics that John Baez, a mathematical physicist at UC Riverside who's become a promoter of TCS, pointed to recently include "cryptographic hash functions, pseudo-random number generators, and the amazing theorem of Razborov and Rudich which says roughly that if P is not equal to NP, then this fact is hard to prove." (If you know what P and NP mean, then this last one probably doesn't seem that surprising, but that means you're thinking about it in the wrong direction!) In fact, the question of P versus NP may even have something to say about the kind of self-consistency we can expect in the laws of physics, and whether we can ever hope to find a Grand Unified Theory. (For those of you hoping for worm-hole-based FTL travel in the future, P vs. NP now concerns you, too.)
Alas my enthusiasm for these implications and connections is stunted by a developing cynicism, not because of a failure to deliver on its founding promises (as, for instance, was the problem that ultimately toppled artificial intelligence), but rather because of its inability to convince not just the funding agencies like NSF that it matters, but its inability to convince the rest of Computer Science that it matters. That is, TCS is a vitally important, but a needlessly remote, field of CS, and is valued by the rest of CS for reasons analogous to those for which CS is valued by other disciplines: its ability to get things done, i.e., actual algorithms. This problem is aggravated by the fact that the mathematical training necessary to build toward a career in TCS is not a part of the standard CS curriculum (I mean at the undergraduate level, but the graduate one seems equally faulted). Instead, you acquire that knowledge by either working with the luminaries of the field (if you end up at the right school), or by essentially picking up the equivalent of a degree in higher mathematics (e.g., analysis, measure theory, abstract algebra, group theory, etc.). As Chazelle puts it in his pre-talk interview, "Computer science ... is messy and infuriatingly complex." I argue that this complexity is what makes CS, and particularly TCS, inaccessible and hard-to-appreciated. If Computer Science as a discipline wants to survive to see the "revolution" Chazelle forecasts, it needs to reevaluate how it trains its future members, what it means to have a science of computers, and even further, what it means to have a theory of computers (a point CS does abysmally on). No computer scientist likes to be told her particular area of study is glorified programming, but without significant internal and external restructuring, that is all Computer Science will be to the rest of the world.
posted February 21, 2006 12:06 AM in Scientifically Speaking | permalink | Comments (0)
February 01, 2006
Defending academic freedom
Michael Bérubé, a literature and culture studies professor at Penn. State University, has written a lecture (now an essay) on the academic freedom of the professoriat and the demands by (radical right) conservatives to demolish it, through state-oversight, in the name of... academic freedom. The Medium Lobster would indeed be proud.
As someone who believes deeply in the importance of the free pursuit of intellectual endeavors, and who has a strong interest in the institutions that facilitate that path (understandable given my current choice of careers), Bérubé's commentary resonated strongly with me. Primarily, I just want to advertise Bérubé's essay, but I can't help but editorialize a little. Let's start with the late Sidney Hook, a liberal who turned staunchly conservative as a result of pondering the threat of Communism, who wrote in his 1970 book Academic Freedom and Academic Anarchy that
The qualified teacher, whose qualifications may be inferred from his acquisition of tenure, has the right honestly to reach, and hold, and proclaim any conclusion in the field of his competence. In other words, academic freedom carries with it the right to heresy as well as the right to restate and defend the traditional views. This takes in considerable ground. If a teacher in honest pursuit of an inquiry or argument comes to a conclusion that appears fascist or communist or racist or what-not in the eyes of others, once he has been certified as professionally competent in the eyes of his peers, then those who believe in academic freedom must defend his right to be wrong—if they consider him wrong—whatever their orthodoxy may be.
That is, it doesn't matter what your political or religious stripes may be, academic freedom is a foundational part of having a free society. At it's heart, Hook's statement is simply a more academic restatement of Voltaire's famous assertion: "I disapprove of what you say, but I will defend to the death your right to say it." In today's age of unblinking irony (e.g., Bush's "Healthy Forests" initiative) for formerly shameful acts of corruption, cronyism and outright greed, such sentiments are depressingly rare.
Although I had read a little about the radical right's effort to install affirmative action for conservative professors in public universities (again, these people have no sense of irony), what I didn't know about is the national effort to introduce legislation (passed into law in Pennsylvania and pending in more than twenty other states) that gives the state oversight ability of the contents of the classroom, mostly by allowing students (non-experts) to sue professors (experts) for introducing controversial material in the classroom. Thus, the legislature and the courts (non-experts) would be able to define what is legally permissible classroom content, by clarifying the legal term "controversial", rather than professors (experts). Bérubé:
When [Ohio state senator Larry Mumper] introduced Senate Bill 24 [which allows students to sue professors, as described above] last year, he was asked by a Columbus Dispatch reporter what he would consider 'controversial matter' that should be barred from the classroom. "Religion and politics, those are the main things," he replied.
All I can say in response is that college is not a kind of dinner party. It can indeed be rude to bring up religion or politics at a dinner party, particularly if you are not familiar with all the guests. But at American universities, religion and politics are two of the hundreds of things we discuss on a daily basis. It really is part of our job, even — or especially — if some of us have unpopular opinions on those subjects.
How else do we learn but by having our pre- and misconceptions challenged by those people who have studied these things, been trained by other experts and been recognized by their peers as an authority? Without academic freedom as defined by Hook and defended by Bérubé, a university degree will signify nothing more than having received the official State-sanctioned version of truth. Few things would be more toxic to freedom and democracy.
posted February 1, 2006 10:45 PM in Simply Academic | permalink | Comments (0)
December 19, 2005
On modeling the human response time function; Part 3.
Much to my surprise, this morning I awoke to find several emails in my inbox apparently related to my commentary on the Barabasi paper in Nature. This morning, Anders Johansen pointed out to myself and Luis Amaral (I can only assume that he has already communicated this to Barabasi) that in 2004 he published an article entitled Probing human response times in Physica A about the very same topic using the very same data as that of Barabasi's paper. In it, he displays the now familiar heavy-tailed distribution of response times and fits a power law of the form P(t) ~ 1/(t+c) where c is a constant estimated from the data. Asymptotically, this is the same as Barabasi's P(t) ~ 1/t; it differs in the lower tail, i.e., for t < c where it scales more uniformly. As an originating mechanism, he suggests something related to a spin-glass model of human dynamics.
Although Johansen's paper raises other issues, which I'll discuss briefly in a moment, let's step back and think about this controversy from a scientific perspective. There are two slightly different approaches to modeling that are being employed to understand the response-time function of human behavior. The first is a purely "fit-the-data" approach, which is largely what Johansen has done, and certainly what Amaral's group has done. The other, employed by Barabasi, uses enough data analysis to extract some interesting features, posits a mechanism for the origin of those and then sets about connecting the two. The advantage of developing such a mechanistic explanation is that (if done properly) it provides falsifiable hypotheses and can move the discussion past simple data-analysis techniques. The trouble begins, as I've mentioned before, when either a possible mechanistic model is declared to be "correct" before being properly vetted, or when an insufficient amount of data analysis is done before positing a mechanism. This latter kind of trouble allows for a debate over how much support the data really provides to the proposed mechanism, and is exactly the source of the exchange between Barabasi et al. and Stouffer et al.
I tend to agree with the idea implicitly put forward by Stouffer et al.'s comment that Barabasi should have done more thorough data analysis before publishing, or alternatively, been a little more cautious in his claims of the universality of his mechanism. In light of Johansen's paper and Johansen's statement that he and Barabasi spoke at the talk in 2003 where Johansen presented his results, there is now the specter that either previous work was not cited that should have been, or something more egregious happened. While not to say that this aspect of the story isn't an important issue in itself, it is a separate one from the issues regarding the modeling, and it is those with which I am primarily concerned. But, given the high profile of articles published in journals like Nature, this kind of gross error in attribution does little to reassure me that such journals are not aggravating certain systemic problems in the scientific publication system. This will probably be a topic of a later post, if I ever get around to it. But let's get back to the modeling questions.
Seeking to be more physics and less statistics, the ultimate goal of such a study of human behavior should be to understand the mechanism at play, and at least Barabasi did put forward and analyze a plausible suggestion there, even if a) he may not have done enough data analysis to properly support it or his claims of universality, and b) his model assumes some reasonably unrealistic behavior on the part of humans. Indeed, the former is my chief complaint about his paper, and why I am grateful for the Stouffer et al. comment and the ensuing discussion. With regard to the latter, my preference would have been for Barabasi to have discussed the fragility of his model with respect to the particular assumptions he describes. That is, although he assumes it, humans probably don't assign priorities to their tasks with anything like a uniformly random distribution and nor do humans always execute their highest priority task next. For instance, can you decide, right now without thinking, what the most important email in your inbox is at this moment? Instead, he commits the crime of hubris and neglects these details in favor of the suggestiveness of his model given the data. On the other hand, regardless of their implausibility, both of these assumptions about human behavior can be tested through experiments with real people and through numerical simulation. That is, these assumptions become predictions about the world that, if they fail to agree with experiment, would falsify the model. This seems to me an advantage of Barabasi's mechanism over that proposed by Johansen, which, by relying on a spin glass model of human behavior, seems quite trickier to falsify.
But let's get back to the topic of the data analysis and the argument between Stouffer et al. and Barabasi et al. (now also Johansen) over whether the data better supports a log-normal or a power-law distribution. The importance of this point is that if the log-normal is the better fit, then the mathematical model Barabasi proposes cannot be the originating mechanism. From my experience with distributions with heavy tails, it can be difficult to statistically (let alone visually) distinguish between a log-normal and various kinds of power laws. In human systems, there is almost never enough data (read: orders of magnitude) to distinguish these without using standard (but sophisticated) statistical tools. This is because for any finite sample of data from an asymptotic distribution, there will be deviations that will blur the functional form just enough to look rather like the other. For instance, if you look closely at the data of Barabasi or Johansen, there are deviations from the power-law distribution in the far upper tail. Stouffer et al. cite these as examples of the poor fit of the power law and as evidence supporting the log-normal. Unfortunately, they could simply be due to deviations due to finite-sample effects (not to be confused with finite-size effects), and the only way to determine if they could have been is to try resampling the hypothesized distribution and measuring the sample deviation against the observed one.
The approach that I tend to favor for resolving this kind of question combines a goodness-of-fit test with a statistical power test to distinguish between alternative models. It's a bit more labor-intensive than the Bayesian model selection employed by Stouffer et al., but this approach offers, in addition to others that I'll describe momentarily, the advantage of being able to say that, given the data, neither model is good or that both models are good.
Using Monte Carlo simulation and something like the Kolmogorov-Smirnov goodness-of-fit test, you can quantitatively gauge how likely a random sample drawn from your hypothesized function F (which can be derived using maximum likelihood parameter estimation or by something like a least-squares fit; it doesn't matter) will have a deviation from F at least as big as the one observed in the data. By then comparing the deviations with an alternative function G (e.g., a power law versus a log-normal), you get a measure of the power of F over G as an originating model of the data. For heavy-tailed distributions, particularly those with a sample-mean that converges slowly or never at all (as is the case for something like P(t) ~ 1/t), sampling deviations can cause pretty significant problems with model selection, and I suspect that the Bayesian model selection approach is sensitive to these. On the other hand, by incorporating sampling variation into the model selection process itself, one can get an idea of whether it is even possible to select one model over another. If someone were to use this approach to analyze the data of human response times, I suspect that the pure power law would be a poor fit (the data looks too curved for that), but that the power law suggested in Johansen's paper would be largely statistically indistinguishable from a log-normal. With this knowledge in hand, one is then free to posit mechanisms that generate either distribution and then proceed to validate the theory by testing its predictions (e.g., its assumptions).
So, in the end, we may not have gained much in arguing about which heavy-tailed distribution the data likely came from, and instead should consider whether or not an equally plausible mechanism for generating the response-time data could be derived from the standard mechanisms for producing log-normal distributions. If we had such an alternative mechanism, then we could devise some experiments to distinguish between them and perhaps actually settle this question like scientists.
As a closing thought, my interest in this debate is not particularly in its politics. Rather, I think this story suggests some excellent questions about the practice of modeling, the questions a good modeler should ponder on the road to truth, and some of the pot holes strewn about the field of complex systems. It also, unfortunately, provides some anecdotal evidence of some systemic problems with attribution, the scientific publishing industry and the current state of peer-review at high-profile, fast turn-around-time journals.
References for those interested in reading the source material.
A. Johansen, "Probing human response times." Physica A 338 (2004) 286-291.
A.-L. Barabasi, "The origin of bursts and heavy tails in human dynamics." Nature 435 (2005) 207-211.
D. B. Stouffer, R. D. Malmgren and L. A. N. Amaral "Comment on 'The origin of bursts and heavy tails in human dynamics'." e-print (2005).
J.-P. Eckmann, E. Moses and D. Sergi, "Entropy of dialogues creates coherent structures in e-mail traffic." PNAS USA 101 (2004) 14333-14337.
A.-L. Barabasi, K.-I. Goh, A. Vazquez, "Reply to Comment on 'The origin of bursts and heavy tails in human dynamics'." e-print (2005).
posted December 19, 2005 04:32 PM in Scientifically Speaking | permalink | Comments (0)
November 27, 2005
Irrational exuberance plus indelible sniping yields delectable entertainment
In a past entry (which sadly has not yet scrolled off the bottom of the front page - sad because it indicates how infrequently I am posting these days), I briefly discussed the amusing public debate by Barabasi et al. and Souffer et al. over Barabasi's model of correspondence. At that point, I found the exchange amusing and was inclined to agree with the response article. However, let me rehash this topic and expose a little more light on the subject.
From the original abstract of the article posted on arxiv.org by Barabasi:
Current models of human dynamics, used from risk assessment to communications, assume that human actions are randomly distributed in time and thus well approximated by Poisson processes. In contrast, ... the timing of many human activities, ranging from communication to entertainment and work patterns, [are] ... characterized by bursts of rapidly occurring events separated by long periods of inactivity. Here we show that the bursty nature of human behavior is a consequence of a decision based queuing process: when individuals execute tasks based on some perceived priority, the timing of the tasks will be heavy tailed, most tasks being rapidly executed, while a few experience very long waiting times.
(Emphasis is mine.) Barabasi is not one to shy away from grand claims of universality. As such, he epitomizes the thing that many of those outside of the discipline hate about physicists, i.e., their apparent arrogance. My opinion is that most physicists accused of intellectual arrogant are misunderstood, but that's a topic for another time.
Stouffer et al. responded a few months after Barabasi's original idea, as published in Nature, with the following (abstract):
In a recent letter, Barabasi claims that the dynamics of a number of human activities are scale-free. He specifically reports that the probability distribution of time intervals tau between consecutive e-mails sent by a single user and time delays for e-mail replies follow a power-law with an exponent -1, and proposes a priority-queuing process as an explanation of the bursty nature of human activity. Here, we quantitatively demonstrate that the reported power-law distributions are solely an artifact of the analysis of the empirical data and that the proposed model is not representative of e-mail communication patterns.
(Emphasis is mine.) In this comment, Stouffer et al. strongly criticize the data analysis that Barabasi uses to argue for the plausibility and, indeed, the correctness of his priority-based queueing model. I admit that when I first read Barabasi's queueing model, I thought that surely the smart folks who have been dealing with queueing theory (a topic nearly a century old!) knew something like this already. Even if that were the case, the idea certainly qualifies as interesting, and I'm happy to see a) the idea published, although Nature was likely not the appropriate place and b) the press attention that Barabasi has brought to the discipline of complex systems and modeling. Anyway, the heart of the data-analysis based critique of Barabasi's work lies in distinguishing two different kinds of heavy-tailed distributions: the log-normal and the power law. Because of a heavy tail is an asymptotic property, these two distributions can be extremely difficult to differentiate when the data only spans a few orders of magnitude (as is the case here). Fortunately, statisticians (and occasionally, myself) enjoy this sort of thing. Stouffer et al. employ such statistical tools in the form of Bayesian model selection to choose between the two hypotheses and find the evidence of the power law lacking. It was quite dissatisfying, however, that Stouffer et al. neglected to discuss their model selection procedure in detail, and instead chose to discuss the politicking over Barabasi's publication in Nature.
And so, it should come as no surprise that a rejoinder from Barabasi was soon issued. With each iteration of this process, the veneer of professionalism cracks away a little more:
[Stouffer et al.] revisit the datasets [we] studied..., making four technical observations. Some of [their] observations ... are based on the authors' unfamiliarity with the details of the data collection process and have little relevance to [our] findings ... and others are resolved in quantitative fashion by other authors.
In the response, Barabasi discusses the details of the dataset that Stouffer et al. fixated on: that the extreme short-time behavior of the data is actually an artifact of the way messages to multiple recipients were logged. They rightly emphasize that it is the existence of a heavy tail that is primarily interesting, rather than its exact form (of course, Barabasi made some noise about the exact form in the original paper). However, it is not sufficient to simply observe a heavy tail, posit an apparently plausible model that produces some kind of such tail and then declare victory, universality and issue a press release. (I'll return to this thought in a moment.) As a result, Barabasi's response, while clarifying a few details, does not address the fundamental problems with the original work. Problems that Stouffer et al. seem to intuit, but don't directly point out.
A month ago, Suresh over at the Geomblog published a comment on the controversy by Michael Mitzenmacher (whose work I greatly enjoy) in which he touches briefly on the real issue here.
While the rebuttal suggests the data is a better fit for the lognormal distribution, I am not a big believer in the fit-the-data approach to distinguish these distributions. The Barabasi paper actually suggested a model, which is nice, although the problem of how to verify such a model is challenge... This seems to be the real problem. Trust me, anyone can come up with a power law model. The challenge is figuring out how to show your model is actually right.
That is, first and foremost, the bursty nature of human activity is odd and, in that alluring voice only those fascinated by complex systems can hear, begs for an explanation. Second, a priority-based queueing process is merely one possible explanation (out of perhaps many) for the heaviness and burstiness. The emphasis is to point out that there is a real difficulty in nailing down causal mechanisms in human systems. often the best we can do is concoct a theory and see if the data supports it. That is, it is exceedingly difficult to go beyond mere plausibility without an overwhelming weight of empirical evidence and, preferably, the vetting of falsifiable hypotheses. The theory of natural selection is an excellent example that has been validated by just such a method (and continues to be). Unfortunately, simply looking at the response time statistics for email or letters by Darwin or Einstein, while interesting from the socio-historical perspective, does not prove the model. On the contrary: it merely suggests it.
That is, Barabasi's work demonstrates the empirical evidence (heavy-tails in the response times of correspondence) and offers a mathematical model that generates statistics of a similar form. It does not show causality, nor does it provide falsifiable hypotheses by which it could be invalidated. Barabasi's work in this case is suggestive but not explanatory, and should be judged accordingly. To me, it seems that the contention over the result derives partly from the overstatement of its generality, i.e., the authors claims their model to be explanatory. Thus, the argument over the empirical data is really just an argument about how much plausibility it imparts to the model. Had Barabasi gone beyond suggestion, I seriously doubt the controversy would exist.
Considering the issues raised here, personally, I think it's okay to publish a results that is merely suggestive so long as it is honestly made, diligently investigated and embodies a compelling and plausible story. That is to say that, ideally, authors should discuss the weakness of their model, empirical results and/or mathematical analysis, avoid overstating the generality of the result (sadly, a frequent problem in many of the papers I referee), carefully investigate possible biases and sources of error, and ideally, discuss alternative explanations. Admittedly, this last one may be asking a bit much. In a sense, these are the things I think about when I read any paper, but particularly when I referee something. This thread of thought seems to be fashionable right now, as I just noticed that Cosma's latest post discusses criteria for accepting or rejecting papers in the peer review process.
posted November 27, 2005 05:00 AM in Scientifically Speaking | permalink | Comments (0)
November 06, 2005
Finding your audience
Some time ago, a discussion erupted on Crooked Timber about the ettiquete of interdisciplinary research. This conversation was originally sparked by Eszter Hargittai, a sociologist with a distinct interest in social network analysis, who complained about some physicists working on social networks and failing to appropriately cite previous work in the area. I won't rehash the details, since you can read them for yourself. However, the point of the discussion that is salient for this post is the question of where and how one should publish and promote interdisciplinary work.
Over the better half of this past year, I have had my own journey with doing interdisciplinary research in political science. Long-time readers will know that I'm referring to my work with here, here and here). In our paper (old version via arxiv), we use tools from extremal statistics and physics to think carefully about the nature and evolution of terrorism, and, I think, uncover some interesting properties and trends at the global level. Throughout the process of getting our results published in an appropriate technical venue, I have espoused the belief that it should either go to an interdisciplinary journal or one that political scientists will read. That is, I felt that it should go to a journal with an audience that would both appreciate the results and understand their implications.
This idea of appropriateness and audience, I think, is a central problem for interdisciplinary researchers. In an ideal world, every piece of novel research would be communicated to exactly that group of people who would get the most out of learning about the new result and who would be able to utilize the advance to further deepen our knowledge of the natural world. Academic journals and conferences are a poor approximation of this ideal, but currently they're the best institutional mechanism we have. To correct for the non-idealness of these institutions, academics have always distributed preprints of their work to their colleagues (who often pass them to their own friends, etc.). Blogs, e-print archives and the world wide web in general constitute interesting new developments in this practice and show how the fundamental need to communicate ideas will co-opt whatever technology is available. Returning to the point, however, what is interesting about interdisciplinary research is that by definition it has multiple target audiences to which it could, or should, be communicated. Choosing that audience can become a question of choosing what aspects of the work you think are most important to science in general, i.e., what audience has the most potential to further develop your ideas? For physicists working on networks, some of their work can and should be sent to sociology journals, as its main contribution is in the form of understanding social structure and implication, and sociologists are best able to use these discoveries to explain other complex social phenomena and to incorporate them into their existing theoretical frameworks.
In our work on the statistics of terrorism, Maxwell and I have chosen a compromise strategy to address this question: while we selected general science or interdisciplinary journals to send our first manuscript on the topic, we have simultaneously been making contacts and promoting our ideas in political science so as to try to understand how to further develop these ideas within their framework (and perhaps how to encourage the establishment to engage in these ideas directly). This process has been educational in a number of ways, and recently has begun to bear fruit. For instance, at the end of October, Maxwell and I attended the International Security Annual Conference (in Denver this year) where we presented our work in the second of two panels on terrorism. Although it may have been because we announced ourselves as computer scientists, stood up to speak, used slides and showed lots of colorful figures, the audience (mostly political scientists, with apparently some government folk present as well) was extremely receptive to our presentation (despite the expected questions about statistics, the use of randomness and various other technical points that were unfamiliar to them). This led to several interesting contacts and conversations after the session, and an invitation to the both of us to attend a workshop in Washington DC on predictive analysis for terrorism that will be attended by people from the entire alphabet soup of spook agencies. Also, thanks to the mention of our work in The Economist over the summer, we have similarly been contacted be a handful of political scientists who are doing rigorous quantitative work in a similar vein as ours. We're cautiously optimistic that this may all lead to some fruitful collaborations, and ultimately to communicating our ideas to the people to whom they will matter the most.
Despite the current popularity of the idea of interdisciplinary research (not to be confused with excitement about the topic itself, which would take the form of funding), if you are interested in pursuing a career in it, like many aspects of an academic career, there is little education about its pitfalls. The question of etiquette in academic research deserves much more attention in graduate school than it currently receives, as does its subtopic of interdisciplinary etiquette. Essentially, it is this last idea that lays at the heart of Eszter Hargittai's original complaint about physicists working on social networks: because science is a fundamentally social exercise, there are social consequences for not observing the accepted etiquette, and those consequences can be a little unpredictable when the etiquette is still being hammered out as in the case of interdisciplinary research. For our work on terrorism, our compromise strategy has worked so far, but I fully expect that, as we continue to work in the area, we will need to more fully adopt the mode and convention of our target audience in order to communicate effectively with them.
posted November 6, 2005 01:15 PM in Simply Academic | permalink | Comments (1)
October 27, 2005
Links, links, links.
The title is perhaps a modern variation on Hamlet's famous "words, words, words" quip to Lord Polonius. Some things I've read recently, with mild amounts of editorializing:
Tim Burke (History professor at Swarthmore College) recently discussed (again) his thoughts on the future of academia. That is, why would it take for college costs to actually decrease. I assume this arises at least partially as a result of the recent New York Times article on the ever increasing tuition rates for colleges in this country. He argues that modern college costs rise at least partially as a result of pressure from lawsuits and parents to provide in loco parentis to the kids attending. Given the degree of hand-holding I experienced at Haverford, perhaps the closest thing to Swarthmore without actually being Swat, this makes a lot of sense. I suspect, however, that tuition prices will continue to increase apace for the time being, if only because enrollment rates continue to remain high.
Speaking of high enrollment rates, Burke makes the interesting point
... the more highly selective a college or university is in its admission policies, the more useful it is for an employer as a device for identifying potentially valuable employees, even if the employer doesn’t know or care what happened to the potential employee while he or she was a student.
This assertion belies an assumption about whose pervasiveness I wonder. Basically, Burke is claiming that selectivity is an objective measure of something. Indeed, it is. It's an objective measure of the popularity of the school, filtered through the finite size of a freshman class that the school can reasonably admit, and nothing else. A huge institution could catapult itself higher in the selectivity rankings simply by cutting the number of students it admits.
Barabasi's recent promotion of his ideas about the relationship between "bursty behavior" among humans and our managing a queue of tasks to accomplish continues to generate press. New Scientist and Physics Web both picked the piece of work on Darwin's, Einstein's and modern email-usage communication patterns. To briefly summarize from Barabasi's own paper:
Here we show that the bursty nature of human behavior is a consequence of a decision based queueing process: when individuals execute tasks based on some perceived priority, the timing of the tasks will be heavy tailed, most tasks being rapidly executed, while a few experience very long waiting times.
A.-L. Barabasi (2005) "The origin of bursts and heavy tails in human dynamics." Nature 435, 207.
That is, the response times are described by a power law with exponent between 1.0 and 1.5. Once again, power laws are everywhere. (NB: In the interest of full disclosure, power laws are one focus of my research, although I've gone on record saying that there's something of an irrational exuberance for them these days.) To those of you experiencing power-law fatigue, it may not come as any surprise that last night in the daily arXiv mailing of new work, a very critical (I am even tempted to say scathing) comment on Barabasi's work appeared. Again, to briefly summarize from the comment:
... we quantitatively demonstrate that the reported power-law distributions are solely an artifact of the analysis of the empirical data and that the proposed model is not representative of e-mail communication patterns.
D. B. Stouffer, R. D. Malmgren and L. A. N. Amaral (2005) "Comment on The origin of bursts and heavy tails in human dynamics." e-print.
There are several interesting threads imbedded in this discussion, the main one being on the twin supports of good empirical research: 1) rigorous quantitative tools for data analysis, and 2) a firm basis in empirical and statistical methods to support whatever conclusions you draw with aforementioned tools. In this case, Stouffer, Malmgren and Amaral utilize Bayesian model selection to eliminate the power law as a model, and instead show that the distributions are better described by a log-normal distribution. This idea of the importance of good tools and good statistics is something I've written on before. Cosma Shalizi is a continual booster of these issues, particularly among physicists working in extremal statistics and social science.
And finally, Carl Zimmer, always excellent, on the evolution of language.
[Update: After Cosma linked to my post, I realized it needed a little bit of cleaning up.]
posted October 27, 2005 01:23 AM in Thinking Aloud | permalink | Comments (0)
September 29, 2005
Networks in our nation's capital
This past week, I attended the Statistics on Networks workshop at the National Academies of Science in Washington DC, where I saw many familiar faces and many new ones. In particular, I was very happy to finally meet Jon Kleinberg, John Doyle, Steve Borgatti and my collaborator Dimitris Achlioptas. And it was nice to see Walter Willinger and Chris Wiggins again, both of whom I met at the MSRI workshop on networks earlier this year. And naturally, it was nice to see my collaborator Mark Newman again, even though we correspond pretty regularly. Now that I've distributed the appropriate linkage for the search engines, let me get on with my thoughts.
This workshop was interesting for a couple of reasons. First, the audience contained statisticians, social scientists, computer science/physics people, and engineers/biologists. Certainly the latter two groups presented very different perspectives on networks, with the former being interested in universality properties and random models of networks, while the latter was much more interested in building or decomposing a particular kind or instance of a network. The social scientists present (and there were many of them) seemed to have a nicely balanced perspective on the usefulness of random models, with perhaps a slight leaning toward the computer science/physics side. Naturally, this all made for interesting dinner and wrap-up discussion. For myself, my bias is naturally in the direction of appreciating models that incorporate randomness. However, it's true that when translated through a particular network model, randomness can itself generate structure (e.g., random graphs with power law degree distributions tend to have a densely connected core of high degree vertices, a structure that is a poor model for the core of the internet, where mixing is disassortative). In the case of real world networks, I think random models yield the most benefit when used to explore the space of viable solutions to a particular constraint or control problem. Eve Marder's work (also at the workshop) on small networks of self-regulating neurons (in this case, those of the lobster gut) is a particularly good example of this approach.
Second, although there were very few graduate students in attendance (I counted three, myself included), the environment was friendly, supportive and generally interesting. The workshop coordinators did a good job of inviting people doing interesting work, and I enjoyed just about all of the talks. Finally, it was interesting to see inside the National Academies a little. This institution is the one that fulfills the scientific inquiries of Congress, although I can't imagine this Congress listens to its scientists very much.
posted September 29, 2005 09:43 PM in Simply Academic | permalink | Comments (0)
August 30, 2005
Reliability in the currency of ideas
The grist of the scientific mill is publications - these are the currency that academics use to prove their worth and contributions to society. When I first dreamt of becoming a scientist, I rationalized that while I would gain less materially than certain other careers, I would be contributing to society in a noble way. But what happens to the currency when its reliability is questionable, when the noblesse is in doubt?
A recent paper in the Public Library of Science (PLoS) Medicine by John Ioannidis discusses "Why most published research findings are false" (New Scientist has a lay-person summary available). While Ioannidis is primarily concerned with results in medicine and biochemistry, his criticism of experimental design, experimenter bias and scientific accuracy likely apply to the broad range of disciplines. In his own words,
The probability that a research claim is true may depend on the study power and bias, the number of other studies on the same question, and, importantly, the ratio of true to no relationships among the relationships probed in each scientific field.
Ioannidis argues is that the current reliance upon the statistical significance p-value in only one direction, i.e., is the chance that the observed data is no different than the null hypothesis measured to be less than some threshold (typically, chance less than 1 in 20), is a dangerous precedent as it ignores the influence of research bias (from things such as finite-size effects, hypothesis and test flexibility, pressure to publish significant findings, etc.). Ioannidis goes on to argue that scientists are often careless in ruling out potential biases in data, methodology and even the hypotheses tested, and that replication by independent research groups is the best way of validating research findings as they constitute the most independent kind of trial possible. That is, confirming an already published result is at least as important as the original finding itself. Yet, he also argues that even then, significance may simply represent broadly shared assumptions.
... most research questions are addressed by many teams, and it is misleading to emphasize the statistically significant findings of any single team. What matters is the totality of the evidence. Diminishing bias through enhanced research standards and curtailing of prejudices may also help. However, this may require a change in scientific mentality that might be difficult to achieve.
In the field of complex systems, where arguably there is a non-trivial amount of pressure to produce interesting and, pardon the expression, universal results, Ioannidis's concerns seem particularly relevant. Without beating the dead horse of finding power laws everyone you look, shouldn't we who seek to explain the complexity of the natural (and man-made) world through simple organizing principles be held to exacting standards of rigor and significance? My work as a referee leads me to believe that my chosen field has insufficiently indoctrinated its practitioners as to the importance of experimental and methodological rigor, and of not over-generalizing or over-stating the importance of your results.
Ioannidis, J. P. A. (2005) "Why most published research findings are false." PLoS Med 2(8):e124
posted August 30, 2005 10:13 AM in Simply Academic | permalink | Comments (0)
July 26, 2005
Global patterns in terrorism; part III
Neil Johnson, a physicist at Lincoln College of Oxford University, with whom I've been corresponding about the mathematics of terrorism for several months, has recently put out a paper that considers the evolution of the conflicts in Iraq and Colombia. The paper (on arxiv, here) relies heavily on the work Maxwell Young and I did on the power law relationship between the frequency and severity of terrorist attacks worldwide.
Neil's article, much like our original one, has garnered some attention among the popular press, so far yielding an article at The Economist (July 21st) that also heavily references our previous work. I strongly suspect that there will be more, particularly considering the July 7th terrorist bombings in London, and Britain's continued conflicted relationship with its own involvement in the Iraq debacle.
Given the reasonably timely attention these analyses are garnering, the next obvious step in this kind of work is to make it more useful for policy-makers. What does it mean for law enforcement, for the United Nations, for forward-looking politicians that terrorism (and, if Neil is correct in his conjecture, the future of modern armed geopolitical conflict) has this stable mathematical structure? How should countries distribute their resources so as to minimize the fallout from the likely catastrophic terrorist attacks of the future? These are the questions that scientific papers typically stay as far from as possible - attempting to answer them takes one out of the scientific world and into the world of policy and politics (shark infested waters for sure). And yet, in order for this work to be relevant outside the world of intellectual edification, some sort of venture must be made.
posted July 26, 2005 12:57 AM in Scientifically Speaking | permalink | Comments (0)
May 21, 2005
The inter-disciplinary politics of interdisciplinary research or, "Hey, that was my idea first."
A few days ago, Eszter Hargittai posted a rant on the joint-blog Crooked Timber about the entre of physicists into the subfield in sociology of social networks and her perception of their contributing mostly nothing of value. Her entry was prompted by this paper about the EuroVision Contest. I learned about the entry first when she reproduced it on the social networking listserv SOCNET; a list on which I lurk mostly because I'm too cheap to pay the membership fee and also because I mainly use it as a way to collect journal references for sociology literature. References which I imagine to myself that I'll read or use one day, although given the poor job I'm currently doing at keeping up with the recent papers in my own field, I may realistically never get around to. (This point is salient, and I'll return to it momentarily.) In the ensuing and relatively lively debate in the post's comments section, someone called for and then received attention from friend Cosma Shalizi, who blogs his own thoughts on the subject in his usual lengthy, heavily cross-referenced and edifying way.
Several meta-commentary thoughts come immediately to mind:
1. Cosma's points are extremely thoughtful and are likely right on the money in terms of seeing the merits of both physicists contributions to social sciences and the argument of their reinvention of wheels. Most relevant to the rant about physicists not contributing anything of value to the field of social networks, he gives four excellent and broad examples of how physicists have added to our knowledge.
2. One of these points, which bears rehashing here, is that physicists are not just interested in social networks (it unfortunately illustrates the irony of the sociologists claims of academic injustice that this observation is abscent from their complaints). Physics training, and particularly that of statistical mechanics, the subfield that most physicists interested in social networks hail from, emphasizes that items of inquiry can, to as great an extent as possible, be treated as interchangeable. Thus, complex networks is the idea that social networks are just one kind of network. The progress physicists have made in carving out the field of complex networks has been somewhat spotty, perhaps because of their not knowing entirely how much of statistical mechanics to import and how much of a reliance on numerical simulation is reasonable (this touches on a related point, that there is not a firm consensus on how computational modeling and simulation should be incorporated into science to the same degree that theory and empiricism have been). If they have been arrogant toward other fields in their attempts to do this, then they should be chastised through letters to the editor of the journals that publish the offending articles. With regard to the EuroVision Contest article, Eszter Hargittai and Kieran Healy's best recourse is to write such a letter to Physica A illustrating that the work is not novel.
3. A point which Cosma omits in his list is connection to social network analysis, via complex network analysis, a large body of mathematical techniques from physics such as percolation theory (he does point out the contribution via network epidemiology), group renormalization, random graph theory, ideas of entropy and techniques for modeling dynamic systems. I may be wrong on these contributions, since I will easily admit that I don't read enough sociology literature. (Update: Cosma notes that sociologists and affiliated statisticians were familiar with Erdos-Renyi random graph theory before the physicists came along.)
4. There's a deeper issue at play here, which Cosma has also discussed (his prolificness is truly impressive, even more so given its high quality). Namely, that there are more physicists than there is funding (or interest?) for physics problems. While I was at Haverford, one of my physics professors told me, without a hint of a smile, that in order to get a job in traditional physics, you basically had to work at one of the national laboratories, work at a particle accelerator laboratory, or work in condensed matter physics. None of these seemed particularly appealing, yet the ideas and approaches of physics were. So, it is perhaps entirely expected that similar folks in my position eventually branch out into other fields. This is, after all, the nature of interdisciplinary research, and physicists (along with mathematicians and, to a lesser degree, chemists) seem particularly well-equipped for this kind of adventure. With the rising emphasis among both funding agencies and universities for interdisciplinary research (which may or may not be simply lip-service), the future likelihood of inter-disciplinary ego-bruising seems high.
5. Obviously, in any scientific endeavor, interdisciplinary or otherwise, scientists should understand the literature that came before (I dislike the term "master", because it implies an amount of time-commitment that I think few people can honestly claim to have spent with literature). In my recent referee work for Physical Review E, I have routinely chastised authors for not writing better introductions that leave a reader with a firm understanding of the context (past and present) in which the fundamental questions they seek to address sit. When it comes to interdisciplinary work, these problems are particularly acute; not only do you have multiple bodies of literature to quickly and succinctly review, but you must also do so in a way accessible to the members of the each field. Some (but, by no means, all) physicists are certainly guilty of this when it comes to writing about social networks, as they are prone to reinventing the wheel. The most egregious example of which is the preferential attachment model of Barabasi and Albert, but it can (and should) be argued that this reinvention was extremely valuable, as it helped spark a wide degree of interest in the previous work and has prompted some excellent work on developing that idea since. So, the fundamental question that I think all of we who claim to be interdisciplinary must face and ultimately answer (in a way that can be communicated to future generations of interdisciplinary researchers, many of whom are in college right now) is, What is the most principled and reasonable way, given the constraints on attention, energy, time, knowledge, intelligence, etc., to allocate proper recognition (typically via citations and coauthorships) to previous and on-going work that is relevant to some interdisciplinary effort?
Or, more succinctly, what's the most practical way to mitigate the inter-disciplinary politics of interdisciplinary research while encouraging it to the fullest extent possible? Closely related are questions about adequately evaluating the merit of research that does not fall squarely within the domain of a large enough body of experts for peer-review. As is the question of how academic departments should value interdisciplinary researchers and what role they should fill in the highly compartmentalized and territorial realm of academic disciplines.
Manual TrackBack: Three-toed Sloth
posted May 21, 2005 04:09 PM in Simply Academic | permalink | Comments (1)
April 21, 2005
Social and anti-social
Over the past week, I have attended a workshop in my field. The workshop is relatively small, although the number of people registered is over 100. What's interesting is the degree to which the social structure of the workshop resembles high school. At the core, you have the popular kids, who have known and worked with each other for years. Their primary interest is in seeing their friends and talking about possible new collaborations. Surrounding this inner-circle is a set of groupies, who know the popular kids, but don't quite have the common history to be considered part of it. Surrounding that group is a possibly larger one of people who are just beginning their social climb in this hierarchy. These are often graduate students, or people who are just moving in to the field.
In retrospect, this kind of hierarchy seems entirely natural, especially when you consider that smart/good people have limited time and likely want to work with other known smart/good people than spend the time cultivating new contacts of unknown quality. The trouble, of course, is that the casual preference for old friendships will tend to lead to perceived exclusivity. That is, if no effort is made to keep the social circles open, they will naturally close.
For a while now, I've been mulling over the assumptions and stereotypes of academia/research being either a social or anti-social endeavor. Some recent thoughts: While certainly there are parts of research that are extremely collaboratory, there's a great deal of it where you sit alone, thinking about something that few other people in the world are interested in. The peer-review process is, on the surface, fairly objective, yet the common single-blindedness of the review process makes it easy for reputation to substitute for quality. The job-search venue appears to be at least as much about who you know as about how good your work is - letters of introduction and reference from known people are often enough (or a requirement) to get a job in a specific field. This part would seem to make it harder for interdisciplinary people to get jobs in more traditional departments; something I'm slightly nervous about. And then, the conference world is largely run by pure social dynamics, with all the trappings of high school mixers, albeit obfuscated, unacknowledged or perhaps slightly ameliorated.
This is, of course, not to say that anyone is going to get a "swirly", or have their lunch money taken away from them. Academics are much too polite for that. But in the ultra-rational world of academia, there are certainly equivalents. I can't imagine that the business world is any better, and indeed, may be significantly worse. Perhaps this is just how human organizations operate: selfishly, irrationally and in a largely ad hoc manner...
posted April 21, 2005 12:34 PM in Simply Academic | permalink | Comments (1)
April 15, 2005
Academia trips over own hubris
It was only a matter of time before cheeky computer science students (from MIT, no less), perhaps inspired by the success of the ever witty and popular R. Robot's random blogging, have developed a tool for creating random computer science papers (text, graphs, and citations). One of these random papers was accepted at WMSCI 2005.
What is WMSCI? In the traditionally easy-to-understand language of conference mission statements, it is
an international forum for scientists and engineers, researchers and, consultants, theoreticians and practitioners in the fields of Systemics, Cybernetics and Informatics.
Obviously. Perhaps for an encore, the students should host a randomly generated conference. To add layer upon layer of hubris to the embarrassment, the conference organizers defended their acceptance of the random paper. Academia rarely gets so tangled in its own contradictions...
Update: Lance Fortnow has an interesting take on the random paper: it's equivalent to academic fraud. His readers, however, seem to think that the prank is more akin to a validation of the review process at the SCI conference (which the conference failed).
posted April 15, 2005 02:59 AM in Simply Academic | permalink | Comments (0)
March 05, 2005
Running a conference
For the past eight months, I've been heavily involved in organizing a "mini" conference within my department. Originally hatched as a way to get graduate students to talk to each other about their research (and similarly to make professors aware of research being done by other groups in our department), it was supposed to replace the long dead "graduate tea" series that used to fill the same role on a weekly basis. And so, myself and the other officers of the Computer Science Graduate Student Association decided to try to make this even as realistic a conference as possible, complete with a review committee, research talks, a poster session, a keynote address and all the trim.
After hundreds of hours of work, many meetings, a couple of free lunches (thank you CSGSA), the conference actually happened yesterday, Friday March 4th. We had 60+ attendees and 20+ presenters (about 10 talks, and 15 posters), a keynote address by Orran Krieger from IBM Research in New York, lots of free food courtesy of sponsorship from Sandia National Labs, and generally a really successful mini-conference. We even got a couple of nice emails from the faculty after-the-fact, thanking us for putting on the event. (About half of them showed up at some point (a few even stayed the entire day), and several sent nice apologies for not attending; it would have been nice to have seen all of them show, as a kind of voting-with-their feet support for students and their research. I guess you can't win them all...) Orran said something very nice about the conference while I was chatting with him before the keynote - he said that his graduate department at Toronto would never have had something like this, which brought together so many people from such divergent aspects of computer science. Perhaps we really did do something unusual.
Having been the general chair for the mini-conference, I can safely say that organizing one of these things is a highly non-trivial task. Duh. Mostly, the pain of doing it revolves around coordinating people, setting time-lines and doing basic logistics, since you rely on other people provide the content for the event. Being the general chair is a bit like being a potter - using only your hands, you have to mold a hunk of rapidly rotating wet clay (which basically wants to fly apart and get everything, including you, very messy) into a coherent, balanced and pleasing form, all before the water evaporates... :) For this kind of event, I'm very grateful that I'd done some things very similar in a previous life at Haverford College, when I was deeply involved in the Customs Program (a.k.a., the freshman orientation and residential advising program). It's definitely true that the more of this kind of thing you do, the easier the next one becomes. You're less scatter-brained, less fatigued, less frustrated, more likely to cover all the bases, more likely to manage the micro-crises that always pop-up, more likely to make good logistical decisions, etc. Yet, there is no part of me that wants to do this kind of thing for a living. It's fun occasionally, as a pleasant change of pace, but there is nothing so mind-numbing as logistics and endless massaging of egos to get things done.
In the next few months, I'll be attending both a high-powered workshop at the Mathematical Science Research Institute (MSRI) in Berkeley and the ACM Symposium on Theory of Computing (STOC) in Baltimore. I have much respect for the people who organize these large-scale events, since they can have hundreds of submissions, hundreds of attendees and budgets orders of magnitude larger than ours. But knowing myself, and my apparent complete inability to stay away from organizing things (indeed, I seem to have an almost compulsive desire to reshape my environment to suite my egotistical beliefs/desires), I'm a bit fearful for the day that I'll actually want to organize something so large!
But for now, it's nice to have another small line on my c.v., but more importantly to have added one more interesting life-experience to my history. Next on my list of life-experiences: a two week trip to Japan later this month.
posted March 5, 2005 02:37 PM in Simply Academic | permalink | Comments (1)
January 24, 2005
The Dark Underbelly
Fear and Loathing are not words that you typically associate with people engaged in research. Things like Serious and Measured, or even, for some people, Creative and Dramatic. I recently had a pair of extremely unpleasant experiences, in which the guilty, who shall remain nameless, exhibited all the open-mindedness and aplomb of a jealous and insecure thirteen year old. What on earth causes grown men, established academics no less, to behave like this?
Academic research, although it pretends to be a meritocracy, uses social constructs like reputation, affiliation and social-circles as a short-hand for quality. This is the heart of how we can avoid reading every paper or listening to every presentation with a totally open mind - after all, if someone has produced a lot of good work before, that's probably a pretty good indicator that they'll do it again. "The best predictor of the future is past behavior." Unfortunately, these social constructs eventually become themselves elements of optimization in a competitive system, and some people focus on them in lieu of doing good work. This, I believe, was the root cause of the overt and insulting hostility I experienced.
Ultimately, because everyone has a finite amount of time and energy, you do have to become more choosy about whom you collaborate with and what ideas you push on. But if everyone only ever did things that moved them "up" in these constructs, no one would ever work with anyone else. What's the point of being an intellectual if it's all turf wars and hostility? Shouldn't one work on things that bring pleasure instead of a constant stream of frustration over poor prestige or paranoia over being scooped? Shouldn't the whole point of being supported by the largess of society be to give as much back as possible, even if this means occasionally not being the most famous or not the guy who breaks the big news?
Maybe these guys don't, but I sure do.
posted January 24, 2005 10:58 AM in Rant | permalink | Comments (0)
January 21, 2005
Reality Distortion Fields
Charisma, they may call it. Jealously being their reaction, while their disdain becomes a weapon of their retribution. Such are the slings and arrows of being both successful and unconventional within academia.
Some people (and institutions) are naturally media hounds. They thrive on the attention and, in turn, the attention drives them toward generating more of the same. For people, we call this "drama" and them "drama queens", but for institutions, we don't for some reason. But you have to admire places like the MIT Media Lab, which consistently pursues a radical vision of the future, despite disdain from the more traditional (provincial?) halls of the academy. Unfortunately, this is no surprise considering America's long tradition of love-hate for the people that the famous Chiat/Day advertising campaign for Apple Computer hailed when it said "the people who are crazy enough to think they can change the world are the ones who usually do." The tech boom of the 1990s seemed to suggest a cultural détente between the forces of tradition and the forces of freakdom, but in the increasingly conservative environment of today, we seem less accommodating.
I have been here for a week now, soaking up the cultural vibe that splilleth over so copiously. Surrounded by passionate people, clashing colored facades, ubiquitously snaking computer cables and omnipresent flashing monitors, the Media Lab feels like a perpetual start-up company that never has to go public or grow into a curmudgeonly hierarchy. As I sit now in a third floor office attached to the Borg Lab (a.k.a. the wearable computing lab) , I think I have a sense of what makes this place special, what makes this place tick and why it both deserves and preserves the professional envy it receives. I remember that when I asked one of my professors at my alma mater about the Media Lab, which I was considering for graduate school, he demurred by saying that they were very creative people who often do pretty outlandish research.
Perhaps he didn't realize how accurate he was being - creative and outlandish are exactly what make the Media Lab unique, and exactly what attracts smart students and faculty bent on changing the world. Although they certainly do research, the pretty strange topics they explore could be more accurately described as "creative engineering".
With an emphasis on demo-able projects that can be shown-off to the corporate sponsors who keep the Lab flush with money, it's natural that there is both a degree of competition as to who can have the most flashy demo, and a natural drive toward creating the applications of technology that will define the future. Truly, the Media Lab is an outsourced research and development center, primed with the passions and ambitions of smart people in love with the possibility of changing the world through technology.
posted January 21, 2005 02:00 AM in Simply Academic | permalink | Comments (0)
January 16, 2005
The Democratization of the Academy
While news surfing the Web today, I came across an article on Slate about the decline of the real prestige that an Ivy League education garners within the business world. The article builds off of a recent paper by two Wharton School economists who chart the decline in the number of Ivy League degrees among the business executives in the Fortune 100 over the last 20 years. Although the Slate article is interesting, the paper itself yields some great insights:
"In 2001, ... executives were younger, more likely to be women, and less likely to have been Ivy League educated. Most important, they got to the executive suite about four years faster than in 1980 and did so by holding fewer jobs on the way to the top. (In particular, women in 2001 got to their executive jobs faster than their male counterparts -- there were no women executives in the Fortune 100 list in 1980)."
Although I'm less concerned in general with the business world side of this discussion, it closely mirrors an issue which sometimes seems painfully important to me as a graduate student at a public university that is not considered to be an elite institution. If the business world data supports an ending of the Ivy League hegemony, then one may wonder if the same is also happening within academia itself. Is the meritocratic, yet oddly idealistic dream coming true that one's worth in the academy will be based wholly on the work one has produced and not based on either the institution's name attached to one's resume?
Somehow, I don't think news of this revolution has reached the ears of the hiring committees at the elite institutions, but I'll leave that discussion for another entry. In a narcissistic article published in Physical Review, covered for popular consumption by the New York Times, documents the rise of scientific publications and Nobel prize winners coming from outside the U.S. The self-absorbed U.S. media reported this observation negatively, as being representative of the diminishing pre-eminence of U.S. science. I viewed it more optimistically: it would seem that the world community is becoming more active in science and that we may, in fact, be witnessing the forces of democracy assaulting the ivory towers themselves.
But what are the prospects of a talented, but non-prestigous degree-bearing post-graduate? My advisor frequently tries to deflect my concern about such prospects, saying that in the past 20 to 30 years, a significant trend in academia has been gaining momentum.
During this time, he sagely counsels me, a lot of great people have ended up at places that used to be not so great. And now, it's not so important where you went as much as who you worked for and what you produced.
In support of this egalitarian sentiment, when I served on the faculty search committee in my department in Spring 2004, I observed something surprisingly hopeful. Something which I can only hope is an ascendent practice among hiring committees, although given my own previous experience at a prestigous institution, I'm not sure the forces of democracy have done much to assail bastions of the elite. When we on the search committee looked at a candidate's resume, if they graduated from an elite institution, we applied more strict standards, and generally, considered the list of publications to be paramount to their value.
"Given that they had all these resources available to them, what did they do with their time?", we asked.
"This person was in a really good lab at a really good school, but look at this small/weak publication list".
"This person has great publications," someone would say, without ever mentioning the school they went to.
So, despite occasional bouts of prestige-envy of my fellows at MIT, Yale, Columbia, Berkeley and Stanford, I now nurture the slight optimism that the academy may be maturing into the meritocratic utopia that it pretends to be. Of course, the competing trends of the corporatization of universities and the down-conversion of tenure track positions to part-time adjunct positions may mean this positive note is ultimately squelched before it can become widespread.
posted January 16, 2005 12:35 AM in Simply Academic | permalink | Comments (0)